Article
Big Data Challenges and Opportunities for Disaster Early Warning System
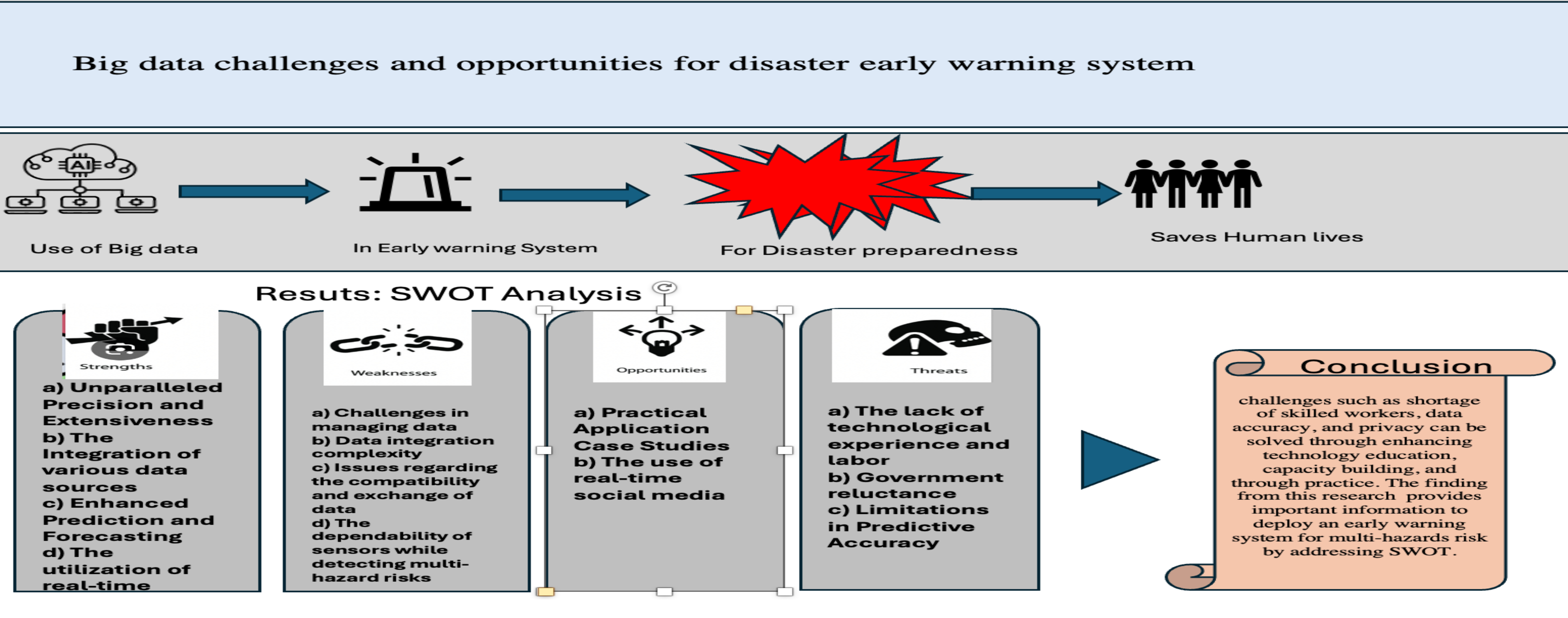
Downloads
Download

This work is licensed under a Creative Commons Attribution 4.0 International License.
Copyright
The authors shall retain the copyright of their work but allow the Publisher to publish, copy, distribute, and convey the work.
License
Prevention and Treatment of Natural Disasters (PTND) publishes accepted manuscripts under Creative Commons Attribution 4.0 International (CC BY 4.0). Authors who submit their papers for publication by Prevention and Treatment of Natural Disasters (PTND) agree to have the CC BY 4.0 license applied to their work, and that anyone is allowed to reuse the article or part of it free of charge for any purpose, including commercial use. As long as the author and original source is properly cited, anyone may copy, redistribute, reuse and transform the content.
The application of big data in early warning systems (EWS) for multi-hazard risk management is becoming increasingly popular due to its enormous potential. Nevertheless, despite noteworthy achievements, there are still several enduring obstacles. This study aims to evaluate the advantages and difficulties through a comprehensive SWOT (strengths, weaknesses, opportunities, threats) analysis and the use of existing literature. An extensive literature analysis was conducted, incorporating perspectives of researchers from diverse background investigating both technical and social elements. The study results showed several notable strengths, such as unmatched accuracy and comprehensiveness, the incorporation of several data sources to improve prediction and forecasting, and the exploitation of up-to-the-minute data. On the other hand, problems, such as insufficient monitoring of hazards and a scarcity of interconnected sensors, especially common in less developed countries, were recognized. Lack of adequate coverage leaves a substantial percentage of the population vulnerable to disaster risks as a result of inadequate early warning systems. Ultimately, although big data offers significant possibilities, its complete capacity remains unexplored in the least developing nations due to existing obstacles, such as shortage of skilled worker, data accuracy and privacy which can be solved by enhancing technology education, capacity building, and practice. The objective of this study is to help such nations identify and overcome underlying challenges by implementing suitable measures.
Keywords:
disaster early warning system (EWS) big data SWOTHighlights
- Extensive literature analysis on Big data and Early warning system
- SWOT analysis integrating diverse perspectives
- Unmatched accuracy and comprehensiveness in hazard prediction
- Improved forecasting through integration of multiple data sources
- Identification of challenges like insufficient hazard monitoring and sensor connectivity
- Recommendations for enhancing technology education and capacity building
References
- Monrat, A.A.; Islam, R.U.; Hossain, M.S.; Andersson, K. Challenges and Opportunities of Using Big Data for Assessing Flood Risks. In Applications of Big Data Analytics: Trends, Issues, and Challenges, 1st ed.; Alani, M.M., Tawfik, H., Saeed, M., Anya, O., Eds.; Springer: Cham, Switzerland, 2018; pp. 31–42.
- Yu, M.; Yang, C.; Li, Y. Big Data in Natural Disaster Management: A Review. Geosciences 2018, 8, 165.
- Stephens-Davidowitz, S. Everybody Lies: What the Internet Can Tell Us About Who We Really Are, 2st ed.; Bloomsbury Publishing: London, UK, 2018; 352p.
- Mayer-Schönberger, V.; Cukier, K. Big data: A Revolution That Will Transform How We Live, Work, and Think. Permissions, Houghton Mifin Harcourt Publishing Company: New York, USA, 2013; 244p.
- Al-Mekhlal, M.; Khwaja, A.A. A Synthesis of Big Data Definition and Characteristics. In Proceedings of the 22nd IEEE International Conference on Computational Science and Engineering and 17th IEEE International Conference on Embedded and Ubiquitous Computing, New York, USA, 1–3 August 2019.
- Liu, J.; Lee, J.; Zhou, R. Review of Big Data and AI Application in Typhoon-Related Disaster Risk Early Warning in Typhoon Committee Region. Trop. Cyclone Res. Rev. 2023, 12, 341–353.
- Intrieri, E.; Raspini, F.; Fumagalli, A.; Lu, P.; Del Conte, S.; Farina, P.; Allievi, J.; Ferretti, A.; Casagli, N. The Maoxian landslide as Seen from Space: Detecting Precursors of Failure with Sentinel-1 Data. Landslides 2018, 15, 123–133.
- Kelevitz, K.; Tiampo, K.F.; Corsa, B.D. Improved Real-Time Natural Hazard Monitoring Using Automated DInSAR Time Series. Remote Sens. 2021, 13, 867.
- Mutanga, O.; Kumar, L. Google Earth Engine Applications. Remote Sens. 2019, 11, 591.
- Khalid, M.A.; Roxin, A.; Cruz, C.; Ginhac, D. A Review on Applications of Big Data for Disaster Management. In Proceedings of the 2017 13th International Conference on Signal-Image Technology & Internet-Based Systems, Jaipur, India, 4–7 December 2017.
- Big Data Analytics in Prevention, Preparedness, Response, and Recovery in Crisis and Disaster Management. Available online: https://www.inase.org/library/2015/zakynthos/bypaper/COMPUTERS/COMPUTERS-78.pdf (accessed on 4 February 2024).
- 2009 Global Assessment Report on Disaster Reduction: Thematic Progress Review Sub-Component on Early Warning Systems. Available online: https://www.preventionweb.net/english/hyogo/gar/2011/en/bgdocs/GAR-2009/background_papers/Chap5/thematic-progress-reviews/WMO-early-warning-systems.doc (accessed on 5 February 2024).
- Monrat, A.A.; Islam, R.U.; Hossain, M.S.; Andersson, K. Challenges and Opportunities of Using Big Data for Assessing Flood Risks. In Applications of Big Data Analytics: Trends, Issues, and Challenges, 1st ed.; Alani, M.M., Tawfik, H., Saeed, M., Anya, O., Eds.; Springer: Cham, Switzerland, 2018; pp. 31–42.
- Rathnasinghe, A.; Kulatunga, U. The potential of Using Big Data for Disaster Resilience: The case of Sri Lanka. In Proceedings of the 8th World Construction Symposium, Colombo, Sri Lanka, 8–10 November 2019.
- Sharath Kumar, C.R.; Praveena K.B. SWOT Analysis. Int. J. Adv. Res. 2023, 11, 744–748.
- Dai, W.; Tang, Y. Ensemble Learning Technology for Coastal Flood Forecasting in Internet-of-Things-Enabled Smart City. Int. J. Comput. Intell. Syst. 2021, 14, 166.
- Sarker, M.N.I.S.; Peng, Y.; Yiran, C.; Shouse, R.C. Disaster Resilience Through Big Data: Way to Environmental Sustainability. Int. J. Disaster Risk Reduct. 2020, 51, 101769.
- The Potential of Earth Observation, Big Data, Artificial Intelligence, and Machine Learning to Improve Financial Risk Management: World Bank Crisis Risk Finance Analytics Strategic Overview. Availabel online: https://documents.worldbank.org/pt/publication/documents-reports/documentdetail/099063023124534173/p172227099e8ef0490a8bd061852b13ae78 (accessed on 5 February 2024).
- Lv, Z.; Li, X.; Choo, K.K.R. E-Government Multimedia Big Data Platform for Disaster Management. Multimedia Tools Appl. 2018, 77, 10077–10089.
- Early Warning Systems for Improving Food Security in East and Southern Africa. Availabel online: https://documents1.worldbank.org/curated/en/104401555444833726/pdf/Early-Warning-Systems-for-Improving-Food-Security-in-East-and-Southern-Africa.pdf (accessed on 4 February 2024).
- Masupha, T.E.; Moelesti, M.E.; Tsubo, M. Prospects of an Agricultural Drought early Warning System in South Africa. Int. J. Disaster Risk Reduct. 2021, 66, 102570.
- Disruptive Technologies for Development (DT4D): Unleashing Innovation in Developing Countries. Availabel online: https://www.worldbank.org/en/events/2021/09/22/disruptive-technologies-for-development-dt4d-unleashing-innovation-in-developing-countries (accessed on 4 February 2024).
- Disaster Management Operations–Big Data Analytics to Resilient Supply Networks. Available online: http://euroma2017.eiasm.org/userfiles/HKJGLML_GDFJMK_AW7YG3VT.pdf (accessed on 5 February 2024).
- Musaev, A.; Wang, D.; Pu, C. LITMUS: A Multi-Service Composition System for Landslide Detection. IEEE Trans. Serv. Comput. 2015, 8, 715–726.
- Zhao, W.; Cheng, Y.; Hou, J.; Chen, Y.; Ji, B.; Ma, L. A Regional Early Warning Model of Geological Hazards Based on Big Data of Real-Time Rainfall. Nat. Hazards 2023, 116, 3465–3480.
- Enenkel, M.; Saenz, S.M.; Dookie, D.S.; Braman, L.; Obradovich, N.; Kryvasheyeu, Y. Social Media Data Analysis and Feedback for Advanced Disaster Risk Management. arXiv 2018, arXiv:1802.02631.
- Jongman, B.; Wagemaker, J.; Revilla Romero, B.; Coughlan de Perez, E. Early Flood Detection for Rapid Humanitarian Response: Harnessing near Real-Time Satellite and Twitter Signals. ISPRS Int. J. Geo-Inf. 2015, 4, 2246–2266.
- 2020 State of Climate Services: Risk Information and Early Warning Systems. Available online: https://library.wmo.int/idurl/4/57191 (accessed on 4 February 2024).
- Shah, S.A.; Seker, D.Z.; Hameed, S.; Draheim, D. The Rising role of Big Data Analytics and IoT in Disaster Management: Recent Advances, Taxonomy and Prospects. IEEE Access 2019, 7, 54595–54614.
- Thirugnanam, H.; Ramesh, M.V.; Rangan, V.P. Enhancing the Reliability of landslide Early Warning Systems by Machine Learning. Landslides 2020, 17, 2231–2246.
- Big Data Interoperability Analysis. Available online: https://joinup.ec.europa.eu/sites/default/files/document/2018-05/SC508DI07171%20D05.02%20Big%20Data%20Interoperability%20Analysis_v1.00.pdf (accessed on 6 February 2024).
- Adi, P.; Prasetya, D.; Setiawan, A.; Nachrowie, N.; Arifuddin, R. Design of Tsunami Detector Based Sort Message Service Using Arduino and SIM900A to GSM/GPRS Module. In Proceedings of the ICASI 2019, Banda Aceh, Indonesia, 18 July 2019.
- Kato, T.; Terada, Y.; Tadokoro, K.; Kinugasa, N.; Futamura, A.; Toyoshima, M.; Yamamoto, S.I.; Ishii, M.; Tsugawa, T.; Nishioka, M.; et al. Development of GNSS Buoy for a Synthetic Geohazard Monitoring System. J. Disaster Res. 2018, 13, 460–471.
- Darmawan, S.; Irawan, B.; Setianingsih, C.; Murty, M.A. Design of detection device for sea water waves with fuzzy algorithm based on internet of things. In Proceedings of the 2020 IEEE International Conference on Industry 4.0, Artificial Intelligence, and Communications Technology, IAICT 2020, Bali, Indonesia, 7–8 July 2020; pp. 75–80.
- Esposito, M.; Palma, L.; Belli, A.; Sabbatini, L.; Pierleoni, P. Recent Advances in Internet of Things Solutions for Early Warning Systems: A Review. Sensors 2022, 22, 2124.
- The Governance of Data in a Digitally Transformed European Society. Available online: https://www.researchgate.net/profile/Marina-Micheli/publication/330223608_The_Governance_of_Data_in_a_Digitally_Transformed_European_Society/links/5c34d84d92851c22a364b547/The-Governance-of-Data-in-a-Digitally-Transformed-European-Society.pdf (accessed on 4 February 2024).
- Yolo, M.; Adeyinka, A.; Mpho, M.; Muthoni, M. A Scalable Semantic Framework for an Integrated Multi-Hazard Early Warning System. In Proceedings of 2023 International Conference on Artificial Intelligence, Big Data, Computing and Data Communication Systems, Durban, South Africa, 3–4 August 2023.
- Khan, A.; Gupta, S.; Gupta, S.K. Multi-Hazard Disaster Studies: Monitoring, Detection, Recovery, and Management, Based on Emerging Technologies and Optimal Techniques. Int. J. Disaster Risk Reduct. 2020, 47, 101642.
- Agbehadji, I.E.; Mabhaudhi, T.; Botai, J.; Masinde, M. A Systematic Review of Existing Early Warning Systems’ Challenges and Opportunities in Cloud Computing Early Warning Systems. Clim. 2023, 11, 118.
- Artificial Intelligence High-Level Expert Group. Ethics Guidelines for Trustworthy AI. European Commission, Brussels: 2019.
- Trogrlić, R.Š.; Homberg, M.V.D.; Budimir, M.; McQuistan, C.; Sneddon, A.; Golding, B. Early Warning Systems and Their Role in Disaster Risk Reduction. In Towards the “Perfect” Weather Warning, 1st ed.; Golding, B., Ed.; Spring: Cham, Switzerland, 2022; pp. 11–46.
- Kafle, S.K. Disaster Early Warning Systems in Nepal: Institutional and Operational Frameworks. J. Geogr. Nat. Disaster 2017, 7, 196.
- Thomalla, F.; Larsen, R.K. Resilience in the Context of Tsunami Early Warning Systems and Community Disaster Preparedness in the Indian Ocean Region. Environ. Hazards 2010, 9, 249–265.
- Big Data Redux: New Issues and Challenges Moving Forward. Available online: http://hdl.handle.net/10125/59546 (accessed on 9 February 2024).
- Dash, B.; Walia, A. Development of Multi-Hazard Early Warning System in India. Disaster risk reduction for resilience, 1st ed.; Eslamian, S., Eslamian, F., Eds.; Springer: Cham, Switzerland, 2022; pp. 99–117.
- Silver, N. The signal and the noise: Why most predictions fail – but some don’t. Penguin: New York, USA, 2012; 544p.
- Hurricane Weather Research and Forecasting (HWRF) Model: 2018 Scientific Documentation. Available online: https://dtcenter.org/sites/default/files/community-code/hwrf/docs/scientific_documents/HWRFv4.0a_ScientificDoc.pdf (accessed on 18 February 2024).
- Yusoff, A.; Din, N.M.; Yussof, S.; Khan, S.U. Big Data Analytics for Flood Information Management in Kelantan, Malaysia. In Proceedings of Student Conference on Research and Development, Kuala Lumpur, Malaysia, 13–14 December 2015.
- Kim, D.; Lee, K.; Hwang-Bo, J.; Kim, H.S.; Kim, S. Development of the Method for Flood Water Level Forecasting and Flood Damage Warning Using an AI-based Model. J. Korean Soc. Hazard Mitigation 2022, 22, 145–156.
- The Role of Big Data in Disaster Management. Available online: https://www.researchgate.net/publication/322265557_The_role_of_big_data_in_disaster_management (accessed on 4 February 2024).