Article
Predicting University Teachers' Behavior Intentions Toward Digital Technologies: An Extension of the Unified Theory of Acceptance and Use of Technology Model (UTAUT)
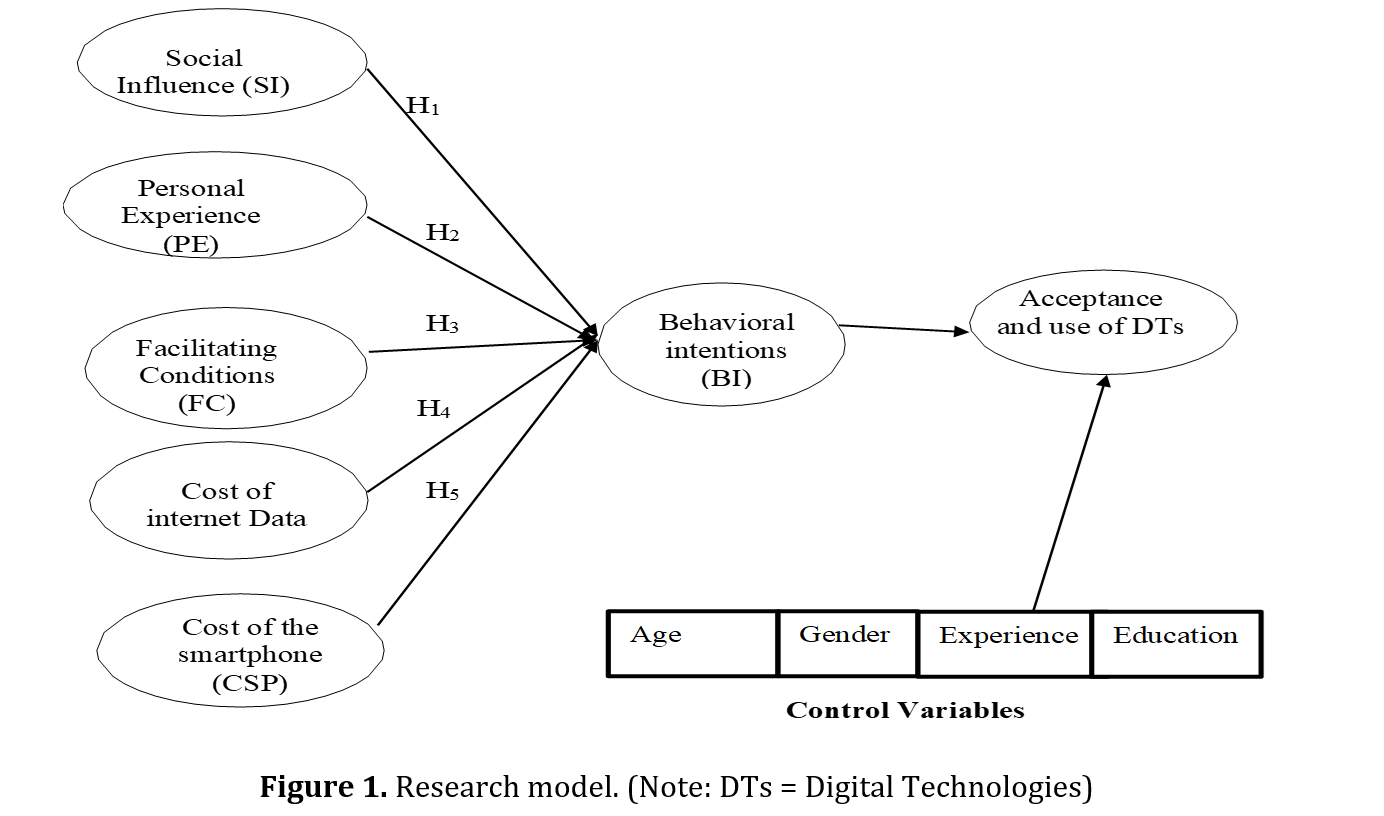
Downloads
Download


This work is licensed under a Creative Commons Attribution 4.0 International License.
Copyright
The authors shall retain the copyright of their work but allow the Publisher to publish, copy, distribute, and convey the work.
License
Journal of Intelligent Communication (JIC) publishes accepted manuscripts under Creative Commons Attribution 4.0 International (CC BY 4.0). Authors who submit their papers for publication by Journal of Intelligent Communication (JIC) agree to have the CC BY 4.0 license applied to their work, and that anyone is allowed to reuse the article or part of it free of charge for any purpose, including commercial use. As long as the author and original source is properly cited, anyone may copy, redistribute, reuse and transform the content.
Information and Communication Technologies over the past decades have enhanced University Teachers’ ability to provide effective and prompt teaching and learning. Therefore, this study explored University Teachers' behaviour intentions toward the use of digital technologies for teaching and learning in higher educational institutions in Ghana. We grounded our study on the Unified Theory of Acceptance and Use of Technology (UTAUT) by testing the contributions of two key variables, the Cost of Internet Data and the Cost of Smart Phones to predict Behaviour Intentions (BI) of university teachers in three higher educational institutions towards the use of Digital Technologies (DTs) for online teaching and learning. We applied Partial Least Square Structural Equation Modelling for data analysis. Hypotheses testing on how the Cost of Internet Data and the Cost of Smartphones influence university teachers' Behaviour Intentions (BI) toward the use of Digital Technologies (DTs) were supported. The findings of our study further showed that university teachers’ intentions to use DTs are influenced by determinants such as social influence, personal experience, and facilitating conditions. The study concludes that the polarity in the findings could help the university authorities to understand the factors to consider in selecting appropriate digital technologies for teaching and learning in universities. The findings from this study are a template for University teachers to get governments to change policies that affect the introduction of digital technologies in higher educational institutions.
Keywords:
information communication digital technologies university teachers UTAUT behavior intentionsReferences
- Rosenbusch, K. Technology intervention: rethinking the role of education and faculty in the transformative digital environment. Adv. Dev. Hum. Resour. 2020, 22(1), 87–101.
- Demuyakor, J. COVID-19 pandemic and higher education: Leveraging on digital technologies and mobile applications for online learning in Ghana. Shanlax Int. J. Educ. 2021, 9(3), 26–38.
- Kumi-Yeboah, A.; Sallar, A.M.; Kiramba, L.K., Kim, Y. Exploring the use of digital technologies from the perspective of diverse learners in online learning environments. Online Learn. 2020, 24(4), 42–63.
- Wang, Q. Modern education technology changed traditional adult teaching mode. Front. High. Educ. 2019, 1(1), 22–26.
- Abbad, M.M. Using the UTAUT model to understand students’ usage of e-learning systems in developing countries. Educ. Inf. Technol. 2021, 26(6), 7205–7224.
- Akbar, M. Digital technology shaping teaching practices in higher education. Front. ICT 2016, 3, 158395.
- Sharma, L.; Srivastava, M. Teachers’ motivation to adopt technology in higher education. J. Appl. Res. High. Educ. 2019, 12(4), 673–692.
- Valverde-Berrocoso, J.; Fernández-Sánchez, M.R.; Revuelta Dominguez, F.I.; Sosa-Díaz, M.J. The educational integration of digital technologies preCovid-19: Lessons for teacher education. PLOS One 2021, 16(8), e0256283.
- Nordhoff, S.; Louw, T.; Innamaa, S.; Lehtonen, E.; Beuster, A.; Torrao, G.; Bjorvatn, A.; Kessel, T.; Malin, F.; Happee, R.; Merat, N. Using the UTAUT2 model to explain public acceptance of conditionally automated (L3) cars: A questionnaire study among 9,118 car drivers from eight European countries. Transp. Res. Part F Traffic Psychol. Behav. 2020, 74, 280–297.
- Venkatesh, V.; Morris, M.G.; Davis, G.B.; Davis, F.D. User acceptance of information technology: Toward a unified view. MIS Q. 2003, 27(3), 425–478.
- Chao, C.M. Factors determining the behavioral intention to use mobile learning: An application and extension of the UTAUT model. Front. Psychol. 2019, 10, 446627.
- Shamizadeh, T.; Jahangiry, L.; Sarbakhsh, P.; Ponnet, K. Social cognitive theory-based intervention to promote physical activity among prediabetic rural people: a cluster randomized controlled trial. Trials 2019, 20(1), 98.
- Rogers, E.M. Diffusion of Innovations, 3rd ed.; Free Press: New York, USA, 1983.
- Rogers, E.M. Diffusion of Innovations, 5th ed.; Free Press: New York, USA, 2003.
- Davis, F.D. A Technology Acceptance Model for Empirically Testing New End-User Information Systems: Theory and Results. Doctoral Dissertation, Massachusetts Institute of Technology, Cambridge, MA, USA, 20 December 1985. http://hdl.handle.net/1721.1/15192
- Lai, P. The literature review of technology adoption models and theories for the novelty technology. J. Inf. Syst. Technol. Manag. 2017, 14(1), 21–38.
- Rossi, A.N.; Armstrong, J.B. Theory of reasoned action vs. theory of planned behavior: Testing the suitability and sufficiency of a popular behavior model using hunting intentions. Hum. Dimens. Wildl. 1999, 4(3), 40–56.
- Dwivedi, Y.K.; Rana, N.P.; Jeyaraj, A.; Clement, M.; Williams, M.D. Re-examining the unified theory of acceptance and use of technology (UTAUT): Towards a revised theoretical model. Inf. Syst. Front. 2019, 21(3), 719–734.
- Liu, D.; Maimaitijiang, R.; Gu, J.; Zhong, S.; Zhou, M.; Wu, Z.; Luo, A., Lu, C.; Hao, Y. Using the unified theory of acceptance and use of technology (UTAUT) to investigate the intention to use physical activity apps: Cross-sectional survey. JMIR mHealth uHealth 2019, 7(9), e13127.
- Zuiderwijk, A.; Janssen, M.; Dwivedi, Y.K. Acceptance and use predictors of open data technologies: Drawing upon the unified theory of acceptance and use of technology. Gov. Inf. Q. 2015, 32(4), 429–440.
- Gu, D.; Khan, S.; Khan, I.U.; Khan, S.U.; Xie, Y.; Li, X.; Zhang, G. Assessing the adoption of e-health technology in a developing country: An extension of the UTAUT model. SAGE Open 2021, 11(3), 21582440211027565.
- Venkatesh, V.; Davis, F.D. A model of the antecedents of perceived ease of use: Development and test. Decis. Sci. 1996, 27(3), 451–481.
- Venkatesh V.; Davis, F.D. A theoretical extension of the technology acceptance model: Four longitudinal field studies. Manage. Sci. 2000, 46(2), 186–204.
- Venkatesh, V.; Thong, J.Y.; Xu, X. Unified theory of acceptance and use of technology: A synthesis and the road ahead. J. Assoc. Inf. Syst. 2016, 17(5), 328–376.
- Mobile broadband costs are keeping developing nations on the fringes of the global Internet economy. Available online: https://www.telecomtv.com/content/green-network/mobile-broadband-costs-are-keeping-developing-nations-on-the-fringes-of-the-global-internet-economy-40993/ (accessed on August 29 2021).
- Average price for 1GB of mobile data in Africa as of 2023, by country. Available online: https://www.statista.com/statistics/1180939/average-price-for-mobile-data-in-africa/ (accessed on August 29 2021).
- Hussain, S.; Fangwei, Z.; Siddiqi, A.F.; Ali, Z.; Shabbir, M.S. Structural equation model for evaluating factors affecting quality of social infrastructure projects. Sustainability 2018, 10(5), 1415.
- Bagozzi R.P.; Yi, Y. On the evaluation of structural equation models. J. Acad. Mark. Sci. 1988, 16(1), 74–94.
- Camilleri, M. Measuring the Students’ Acceptance and Use of Mobile Learning Technologies. Thesis of M.Sc. Educational Leadership and Management, Master-University of Malta, Malta, September 2019.
- Orcan, F. Parametric or non-parametric: Skewness to test normality for mean comparison. Int. J. Assess. Tools Educ. 2020, 7(2), 255–265.
- Cain, M.K.; Zhang, Z.; Yuan, K.H. Univariate and multivariate skewness and kurtosis for measuring nonnormality: Prevalence, influence and estimation. Behav. Res. Methods 2017, 49(5), 1716–1735.
- Shaheen, F.; Ahmad, N.; Waqas, M.; Waheed, A.; Farooq, O. Structural equation modeling (SEM) in social sciences & medical research: a guide for improved analysis. Int. J. Acad. Res. Bus. Soc. Sci. 2017, 7(5), 132–143.
- Perneger, T.V.; Courvoisier, D.S.; Hudelson, P.M.; Gayet-Ageron, A. Sample size for pre-tests of questionnaires. Qual. Life Res. 2015, 24(1), 147–151.
- Patton, M.Q. Qualitative Research & Evaluation Methods: Integrating Theory and Practice, 4th ed.; Sage Publications: Los Angeles, USA, 2015.
- Creswell, J.W. Reflections on the MMIRA the future of mixed methods task force report. J. Mix. Methods Res. 2016, 10(3), 215–219.
- Tesser, A.; Krauss, H. On validating a relationship between constructs. Educ. Psychol. Meas. 1976, 36(1), 111–121.
- Schukat, S.; Heise, H. Towards an understanding of the behavioral intentions and actual use of smart products among German farmers. Sustainability 2021, 13(12), 6666.
- Williams, M.D.; Rana, N.P.; Dwivedi, Y.K. The unified theory of acceptance and use of technology (UTAUT): a literature review. J. Enterp. Inf. Manag. 2015, 28(3), 443–488.
- Safi, S.; Thiessen, T.; Schmailzl, K.J. Acceptance and resistance of new digital technologies in medicine: qualitative study. JMIR Res. Protoc. 2018, 7(12), e11072.
- Vannoy, S.A.; Palvia, P. The social influence model of technology adoption. Commun. ACM 2010, 53(6), 149–153.
- Talukder, M.; Quazi, A. The impact of social influence on individuals' adoption of innovation. J. Organ. Comput. Electron. Commer. 2011, 21(2), 111–135.
- Zalat, M.M.; Hamed, M.S.; Bolbol, S.A. The experiences, challenges, and acceptance of e-learning as a tool for teaching during the COVID-19 pandemic among university medical staff. PLOS One 2021, 16(3), e0248758.
- Phichitchaisopa, N.; Naenna, T. Factors affecting the adoption of healthcare information technology. EXCLI J. 2013, 12, 413.