Article
Effective Analytical Techniques for the Condition Monitoring of Induction Motors
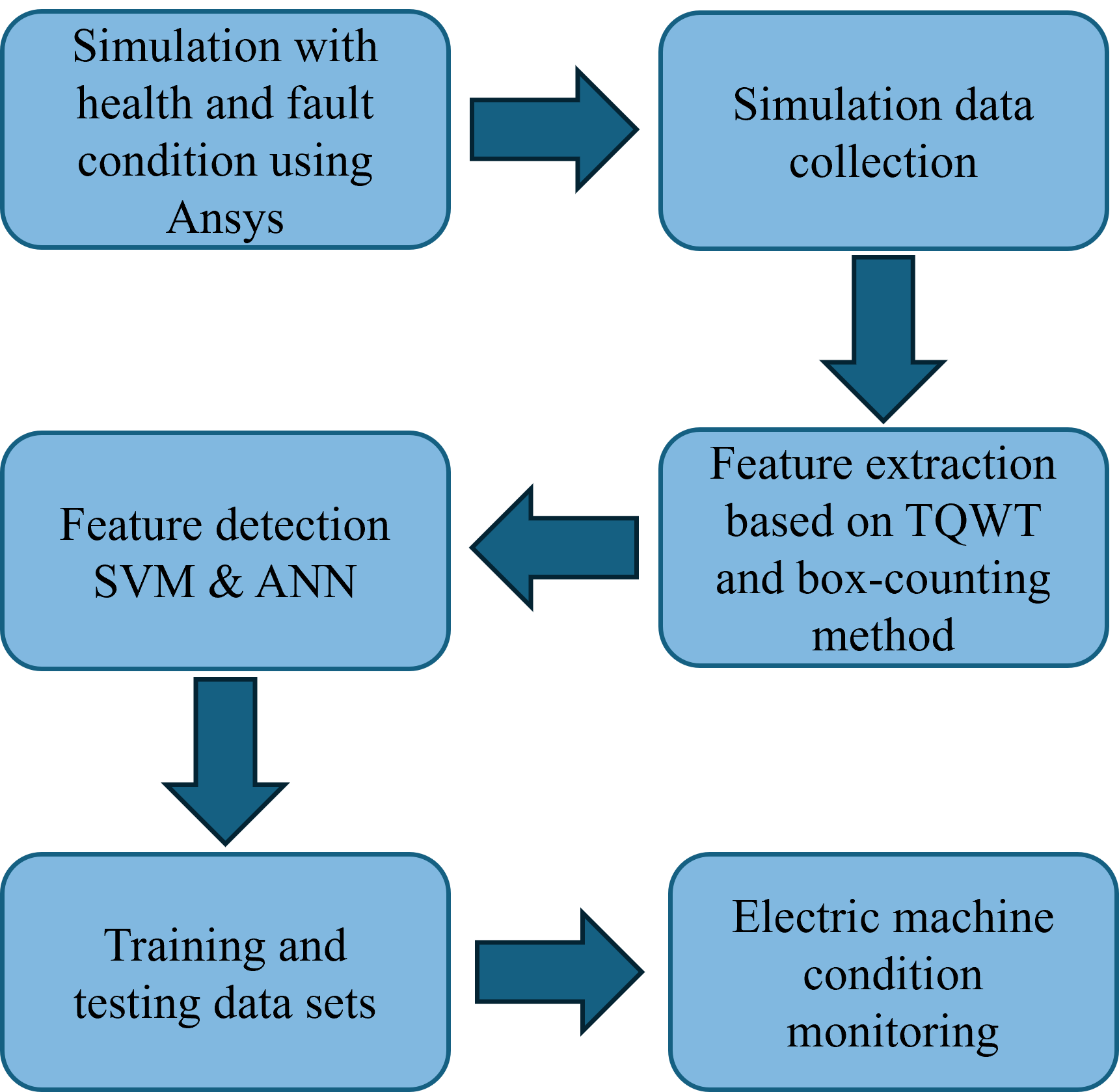
Downloads
Download



This work is licensed under a Creative Commons Attribution 4.0 International License.
Copyright
The authors shall retain the copyright of their work but allow the Publisher to publish, copy, distribute, and convey the work.
License
Digital Technologies Research and Applications (DTRA) publishes accepted manuscripts under Creative Commons Attribution 4.0 International (CC BY 4.0). Authors who submit their papers for publication by DTRA agree to have the CC BY 4.0 license applied to their work, and that anyone is allowed to reuse the article or part of it free of charge for any purpose, including commercial use. As long as the author and original source are properly cited, anyone may copy, redistribute, reuse, and transform the content.
As industrialisation progresses, electric motors are increasingly utilised in manufacturing sectors, and their regular operation plays a crucial role in enhancing production efficiency, safety, and ease. Consequently, there's a growing emphasis on developing technology for monitoring the condition of electric motors. This study focuses on the analysis of common issues like rotor bar failure and eccentricity in induction motors, examining their causes, creating motor models in both normal and malfunctioning conditions through computer simulations, identifying the stator current signals, and comparing their spectra to validate the stator current data. Additionally, this research offers a dependable and efficient dataset for further analysis. The complex and fluctuating nature of the current signals in induction motors necessitates the use of advanced techniques like the tunable-Q wavelet transform (TQWT) and box dimension method for feature extraction, which is more effective in signal characterisation than other approaches. The study then explores the application of Support Vector Machines (SVM) and Artificial Neural Networks (ANN) in fault diagnosis, achieving accuracies of 91.67% and 100%, respectively. The findings indicate that ANN is superior to SVM and suggest this strategy for the automatic detection of motor faults. Implementing such intelligent systems can prevent unexpected and unplanned production interruptions caused by electric motor failures.
Keywords:
Condition monitoring; Induction motors; Finite element analysis; Wavelet transform; Artificial Neural Network; Support Vector Machine
References
- Konar, P.; Chattopadhyay, P. Bearing fault detection of induction motor using wavelet and Support Vector Machines (SVMs). Appl. Soft Comput. 2011, 11, 4203–4211.
- Gangsar, P.; Tiwari, R. Signal based condition monitoring techniques for fault detection and diagnosis of induction motors: A stateoftheart review. Mech. Syst. Signal Process. 2020, 144, 106908.
- O’Donnell, P.; Heising, C.; Singh, C.; Wells, S. Report of large motor reliability survey of industrial and commercial installations. iii. IEEE Trans. Ind. Appl. 1987, 23, 153–158.
- Choudhary, A.; Goyal, D.; Shimi, S.L.; Akula, A. Condition Monitoring and Fault Diagnosis of Induction Motors: A Review. Arch. Comput. Methods Eng. 2019, 26, 1221–1238.
- Siddique, A.; Yadava, G.; Singh, B. A Review of Stator Fault Monitoring Techniques of Induction Motors. IEEE Trans. Energy Convers. 2005, 20, 106–114.
- Sarma, N.; Melecio, J.; Mohammed, A.; Djurovic, S.; Tshiloz, K. An experimental study of winding fault induced slot harmonic effects in the cage rotor induction machine stator current. In Proceedings of the 8th IET International Conference on Power Electronics, Machines and Drives (PEMD 2016), Glasgow, UK, 19–21 April 2016.
- Pillay, P.; Xu, Z. Motor current signature analysis. In Proceedings of the IAS’96. Conference Record of the 1996 IEEE Industry Applications Conference ThirtyFirst IAS Annual Meeting, San Diego, CA, USA,6–10 October 1996; IEEE: Piscataway, NJ, USA, 1996; Volume 1, pp. 587–594.
- Granda, D.; Aguilar, W.G.; ArcosAviles, D.; Sotomayor, D. Broken Bar Diagnosis for Squirrel Cage Induction Motors Using Frequency Analysis Based on MCSA and Continuous Wavelet Transform. Math. Comput. Appl. 2017, 22, 30.
- Vanraj; Goyal, D.; Saini, A.; Dhami, S.S.; Pabla, B.S. Intelligent predictive maintenance of dynamic systems using condition monitoring and signal processing techniques—A review. In Proceedings of the 2016 International Conference on Advances in Computing, Communication, & Automation (ICACCA) (Spring), Dehradun, India, 8–9 April 2016; pp. 1–6.
- Kudelina, K.; Vaimann, T.; Asad, B.; Rassolkin, A.; Kallaste, A.; Demidova, G. Trends and challenges in intelligent condition monitoring of electrical machines using machine learning. Appl. Sci. 2021, 11, 2761.
- Tian, Z. An artificial neural network method for remaining useful life prediction of equipment subject to condition monitoring. J. Intell. Manuf. 2009, 23, 227–237.
- Deng, Y.; Ren, Z.; Kong, Y.; Bao, F.; Dai, Q. A Hierarchical Fused Fuzzy Deep Neural Network for Data Classification. IEEE Trans. Fuzzy Syst. 2016, 25, 1006–1012.
- Awad, M.; Khanna, R. Support vector machines for classification. In Efficient Learning Machines: Theories, Concepts, and Applications for Engineers and System Designers; Apress: Berkeley, CA, USA, 2015; pp. 39–66.
- Widodo, A.; Yang, B.S. Wavelet support vector machine for induction machine fault diagnosis based on transient current signal. Expert Syst. Appl. 2007, 35, 307–316.
- Ferkova, Z. Comparison of twophase induction motor modeling in ANSYS Maxwell 2D and 3D program. In Proceedings of the 2014 ELEKTRO, Rajecke Teplice, Slovakia, 19–20 May 2014; pp. 279–284.
- Basak, D.; Tiwari, A.; Das, S.P. Fault diagnosis and condition monitoring of electrical machines—A Review. In Proceedings of the 2006 IEEE International Conference on Industrial Technology, Mumbai, India, 15–17 December 2006; pp. 3061–3066.
- Kong, Y.; Wang, T.; Chu, F. Adaptive TQWT filter based feature extraction method and its application to detection of repetitive transients. Sci. China Technol. Sci. 2018, 61, 1556–1574.
- Liebovitch, L.S.; Toth, T. A fast algorithm to determine fractal dimensions by box counting. Phys. Lett. A 1989, 141, 386–390.
- Baccarini, L.M.R.; Silva, V.V.R.; Menezes, B.R.; Caminhas, W.M. SVM practical industrial application for mechanical faults diagnostic. Expert Syst. Appl. 2011, 38, 6980–6984.
- Pöyhönen, S. Support Vector Machine Based Classi; Helsinki University of Technology: Espoo, Finland, 2004.
- Sharma, A.; Mathew, L.; Chatterji, S.; Goyal, D. Artificial Intelligence-Based Fault Diagnosis for Condition Monitoring of Electric Motors. Int. J. Pattern Recognit. Artif. Intell. 2020, 34, 2059043.
- Yin, Z.; Hou, J. Recent advances on SVM based fault diagnosis and process monitoring in complicated industrial processes. Neurocomputing 2016, 174, 643–650.
- Yang, B.S.; Han, T.; Yin, Z.J. Fault diagnosis system of induction motors using feature extraction, feature selection and classiJSME Int. J. Ser. C Mech. Sys. Mach. Elem. Manuf. 2006, 49, 734–741.
- Long, Z.; Zhang, X.; Zhang, L.; Qin, G.; Huang, S.; Song, D.; Shao, H.; Wu, G. Motor fault diagnosis using attention mechanism and improved adaboost driven by multisensor information. Measurement 2020, 170, 108718.
- Tyagi, C.S. A comparative study of SVM classifiers and artificial neural networks application for rolling element bearing fault diagnosis using wavelet transform preprocessing. Int. J. Mech. Mechatron. Eng. 2008, 2, 904–912.
- Honarvar Shakibaei Asli, B. Analysis and simulations of eccentric rotors of rotating machinery in polar space. World Appl. Sci. J. 2012, 17, 1675–1682.