Article
On the Use of Raman Blood Spectroscopy and Prediction Machines for Enhanced Care of Endometriosis Patients
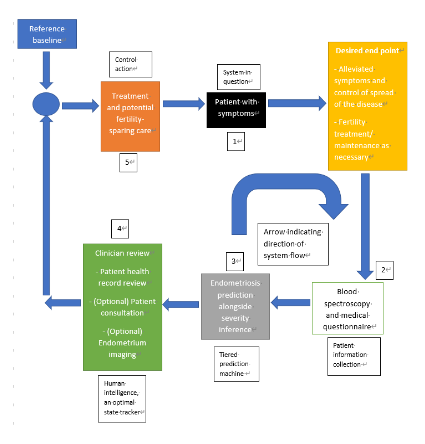
Downloads
Download

This work is licensed under a Creative Commons Attribution 4.0 International License.
Copyright
The authors shall retain the copyright of their work but allow the Publisher to publish, copy, distribute, and convey the work.
License
Digital Technologies Research and Applications (DTRA) publishes accepted manuscripts under Creative Commons Attribution 4.0 International (CC BY 4.0). Authors who submit their papers for publication by DTRA agree to have the CC BY 4.0 license applied to their work, and that anyone is allowed to reuse the article or part of it free of charge for any purpose, including commercial use. As long as the author and original source are properly cited, anyone may copy, redistribute, reuse, and transform the content.
Endometriosis is a prevalent disease of the female endometrium which affects women of all ethnicities and has been seen to be most common in the 25–35 years age group. The disease does not have a definitive cure, hence care and management are the essential components towards dealing with the disease. At present, the predominant means towards the diagnosis of the presence of the disease involves different imaging modalities alongside laparoscopy, where the instrumentation is expensive to acquire and requires clinical expertise. Recently, work has been done by an author who leveraged Raman blood spectroscopy alongside machine learning towards an affordable high throughput means towards the prediction of endometriosis.
This work utilises the Raman blood spectroscopy dataset alongside advanced signal processing, machine learning and clinical cybernetics, towards the design of a prediction machine which sits within a clinical framework to facilitate Human-Machine interaction for an enhanced care strategy for patients with endometriosis. The prediction machine is designed to initially predict whether a patient has the disease, and is then followed by the use of unsupervised learning to form an inference means towards predicting the extent of the disease. The results showed that a combination of the adopted methods could allow for a high prediction of the endometriosis disease. Subsequent work in this area would now include further optimisation of the prediction machine in order to potentially maximise the prediction accuracy.
Keywords:
Machine Learning Public Health Endometriosis Obstetrics and Artificial IntelligenceReferences
- Smolarz B, Szyłło K, Romanowicz H. Endometriosis: Epidemiology, Classification, Pathogenesis, Treatment and Genetics (Review of Literature). Int J Mol Sci 2021;22:10554. https://doi.org/10.3390/ijms221910554.
- Giudice LC. Clinical practice. Endometriosis. N Engl J Med 2010;362:2389–98. https://doi.org/10.1056/NEJMcp1000274.
- Missmer SA, Cramer DW. The epidemiology of endometriosis. Obstet Gynecol Clin North Am 2003;30:1–19, vii. https://doi.org/10.1016/s0889-8545(02)00050-5.
- Parazzini F, Vercellini P, Pelucchi C. Endometriosis: Epidemiology, and Etiological Factors. In: Giudice LC, Evers JLH, Healy DL, editors. Endometriosis: Science and Practice, Wiley-Blackwell; 2012.
- Viganò P, Parazzini F, Somigliana E, Vercellini P. Endometriosis: epidemiology and aetiological factors. Best Pract Res Clin Obstet Gynaecol 2004;18:177–200. https://doi.org/10.1016/j.bpobgyn.2004.01.007.
- Burney RO, Giudice LC. Pathogenesis and pathophysiology of endometriosis. Fertil Steril 2012;98:511–9. https://doi.org/10.1016/j.fertnstert.2012.06.029.
- Bulun SE. Endometriosis. N Engl J Med 2009;360:268–79. https://doi.org/10.1056/NEJMra0804690.
- Olive D, Pritts E. Treatment of Endometriosis. N Engl J Med 2000;345:266–75. https://doi.org/10.1056/NEJM200107263450407.
- Vercellini P, Viganò P, Somigliana E, Fedele L. Endometriosis: pathogenesis and treatment. Nat Rev Endocrinol 2014;10:261–75. https://doi.org/10.1038/nrendo.2013.255.
- Simoens S, Dunselman G, Dirksen C, Hummelshoj L, Bokor A, Brandes I, et al. The burden of endometriosis: costs and quality of life of women with endometriosis and treated in referral centres. Human Reproduction 2012;27:1292–9. https://doi.org/10.1093/humrep/des073.
- Sankaravadivel V, Thalavaipillai S. Symptoms based endometriosis prediction using machine learning. Bulletin of Electrical Engineering and Informatics 2021;10:3102–9. https://doi.org/10.11591/eei.v10i6.3254.
- Raimondo D, Youssef A, Mabrouk M, Del Forno S, Martelli V, Pilu G, et al. Pelvic floor muscle dysfunction on 3D/4D transperineal ultrasound in patients with deep infiltrating endometriosis: a pilot study. Ultrasound Obstet Gynecol 2017;50:527–32. https://doi.org/10.1002/uog.17323.
- Dietz HP. Pelvic floor ultrasound: a review. Am J Obstet Gynecol 2010;202:321–34. https://doi.org/10.1016/j.ajog.2009.08.018.
- Youssef A, Montaguti E, Sanlorenzo O, Cariello L, Salsi G, Morganelli G, et al. Reliability of new three-dimensional ultrasound technique for pelvic hiatal area measurement. Ultrasound Obstet Gynecol 2016;47:629–35. https://doi.org/10.1002/uog.14933.
- Kilpatrick RD, Chiuve SE, Leslie WD, Wegrzyn LR, Gao W, Yang H, et al. Estimating the Effect of Elagolix Treatment for Endometriosis on Postmenopausal Bone Outcomes: A Model Bridging Phase III Trials to an Older Real-World Population. JBMR Plus 2020;4:e10401. https://doi.org/10.1002/jbm4.10401.
- Schipper E, Nezhat C. Video-assisted laparoscopy for the detection and diagnosis of endometriosis: safety, reliability, and invasiveness. Int J Womens Health 2012;4:383–93. https://doi.org/10.2147/IJWH.S24948.
- Vural M. Pelvic pain rehabilitation. Turk J Phys Med Rehabil 2018;64:291–9. https://doi.org/10.5606/tftrd.2018.3616.
- Febbraio MA. Exercise and inflammation. J Appl Physiol (1985) 2007;103:376–7. https://doi.org/10.1152/japplphysiol.00414.2007.
- Koppan A, Hamori J, Vranics I, Garai J, Kriszbacher I, Bodis J, et al. Pelvic pain in endometriosis: painkillers or sport to alleviate symptoms? Acta Physiol Hung 2010;97:234–9. https://doi.org/10.1556/APhysiol.97.2010.2.10.
- Bendifallah S, Puchar A, Suisse S, Delbos L, Poilblanc M, Descamps P, et al. Machine learning algorithms as new screening approach for patients with endometriosis. Sci Rep 2022;12:639. https://doi.org/10.1038/s41598-021-04637-2.
- Pouliakis A, Margari N, Karakitsou E, Valasoulis G, Koufopoulos N, Koureas N, et al. Artificial Intelligence via Competitive Learning and Image Analysis for Endometrial Malignancies: Discriminating Endometrial Cells and Lesions. International Journal of Reliable and Quality EHealthcare (IJRQEH) 2019;8. https://doi.org/10.4018/IJRQEH.2019100102.
- Kleczyk E, Peri A, Yadav T, Komera R, Peri M, Guduru V, et al. Predicting Endometriosis Onset Using Machine Learning Algorithms. 2020. https://doi.org/10.21203/rs.3.rs-135736/v1.
- Akter S, Xu D, Nagel SC, Bromfield JJ, Pelch K, Wilshire GB, et al. Machine Learning Classifiers for Endometriosis Using Transcriptomics and Methylomics Data. Frontiers in Genetics 2019;10.
- Visalaxi S, Muthu TS. Automated prediction of endometriosis using deep learning. International Journal of Nonlinear Analysis and Applications 2021;12:2403–16. https://doi.org/10.22075/ijnaa.2021.5383.
- Lee JH, Kwon S-Y, Chang J, Yuk J-S. Machine Learning Approach to find the relation between Endometriosis, benign breast disease, cystitis and non-toxic goiter. Sci Rep 2019;9:5410. https://doi.org/10.1038/s41598-019-41973-w.
- Parlatan U, Inanc M, Ozgor BY, Oral E, Baştu E, Unlu MB, et al. Raman spectroscopy as a noninvasive diagnostic technique for endometriosis. Scientific Reports 2019. https://doi.org/10.1038/s41598-019-56308-y.
- Wiener N. Cybernetics or control and communication in the animal and the machine. 2. ed., reprint. Cambridge, MA, USA: MIT Press; 2007.
- Nsugbe E, Obajemu O, Samuel OW, Sanusi I. Enhancing care strategies for preterm pregnancies by using a prediction machine to aid clinical care decisions. Machine Learning with Applications 2021;6:100110.
- Nsugbe E. Towards the use of cybernetics for an enhanced cervical cancer care strategy. Intelligent Medicine 2022. https://doi.org/10.1016/j.imed.2022.02.001.
- Jones RR, Hooper DC, Zhang L, Wolverson D, Valev VK. Raman Techniques: Fundamentals and Frontiers. Nanoscale Res Lett 2019;14:231. https://doi.org/10.1186/s11671-019-3039-2.
- Das RS, Agrawal YK. Raman spectroscopy: Recent advancements, techniques and applications. Vibrational Spectroscopy 2011;2:163–76. https://doi.org/10.1016/j.vibspec.2011.08.003.
- Butler HJ, Ashton L, Bird B, Cinque G, Curtis K, Dorney J, et al. Using Raman spectroscopy to characterize biological materials. Nat Protoc 2016;11:664–87. https://doi.org/10.1038/nprot.2016.036.
- Long DA. The Raman effect: a unified treatment of the theory of Raman scattering by molecules. Chichester ; New York: Wiley; 2002.
- Everall N, Hahn T, Matousek P, Parker AW, Towrie M. Photon Migration in Raman Spectroscopy. Appl Spectrosc 2004;58:591–7. https://doi.org/10.1366/000370204774103426.
- Gardiner DJ, Graves PR, Bowley HJ. Practical Raman spectroscopy. Berlin; New York: SpringerVerlag; 1989.
- Nsugbe E. A cybernetic framework for predicting preterm and enhancing care strategies: A review. Biomedical Engineering Advances 2021;2:100024. https://doi.org/10.1016/j.bea.2021.100024.
- Attainable Utopias : First Order Cybernetics. Attainable Utopias n.d. http://attainable-utopias.org/tiki/FirstOrderCybernetics#:~:text=First%20Order%20Cybernetics%20describes%20aspects,its%20whole%20situation%20or%20environment (accessed March 20, 2022).
- Heylighen F, Joslyn C. Second-Order Cybernetics. VubAcBe 2001. http://pespmc1.vub.ac.be/SECORCYB.html (accessed April 21, 2022).