Article
Artificial Intelligence-Assisted Care for Human Newborns with Neurological Impairments
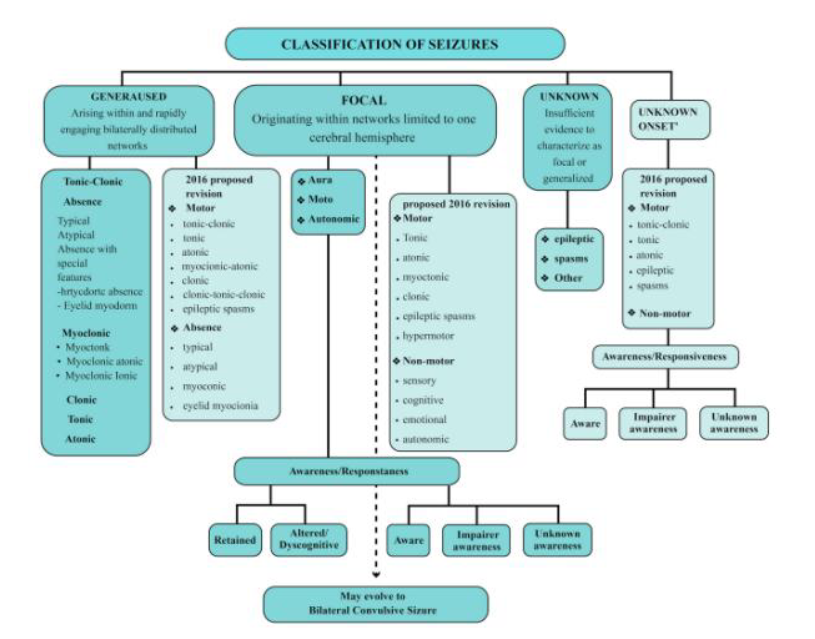
Downloads
Download

This work is licensed under a Creative Commons Attribution 4.0 International License.
Copyright
The authors shall retain the copyright of their work but allow the Publisher to publish, copy, distribute, and convey the work.
License
Digital Technologies Research and Applications (DTRA) publishes accepted manuscripts under Creative Commons Attribution 4.0 International (CC BY 4.0). Authors who submit their papers for publication by DTRA agree to have the CC BY 4.0 license applied to their work, and that anyone is allowed to reuse the article or part of it free of charge for any purpose, including commercial use. As long as the author and original source are properly cited, anyone may copy, redistribute, reuse, and transform the content.
Seizures are a widespread condition affecting 50~65 million people in the world, and newborns are also susceptible to them. EEG is used to monitor the brain activity of newborns with suspected brain injuries, followed by a qualitative waveform interpretation by a group of clinical experts, where the means towards detection of seizures include a set of distinct characteristics in the waveform. This means of seizure detection has been critiqued, particularly due to subjectivity where, at times, waveform reviewing clinicians fail to reach a consensus on the presence of seizure activity in the brain of a newborn. As a means towards dealing with this problem, the author investigated the use of Artificial Intelligence-driven prediction machines capable of an automated diagnosis of seizure, based on a newborn’s EEG waveform. This approach used a reduced selection of EEG electrodes, the Linear Series Decomposition Learner (LSDL), an ensemble of a group of features, and performance comparison across multiple classification models. Secondary work was also carried out, which leveraged the patient information available alongside the EEG dataset. This involved the use of EEG towards predicting the level of asphyxia within the neonatal brain. The results from the seizure prediction exercise showed an increment in prediction performance of the seizures when preprocessed with the LSDL. The results spanned a range of figures (depending on the classification model), with the highest accuracy of 88.1%, while a probabilistic approach towards predicting the extent of seizures provided a maximum accuracy of 93.5%. The results from the secondary analysis showed a maximum accuracy for asphyxia prediction of 89.1%. The obtained results have helped to demonstrate that a reduced selection of electrode segments, alongside the selected algorithms, can serve towards the prediction of seizures for newborns within a neonatal intensive care unit.
Keywords:
Neonates Newborns Artificial intelligence Signal decomposition SVM Machine learningReferences
- Shoeibi, A., Ghassemi, N., Khodatars, M., et al., 2021. Applications of Epileptic Seizures Detection in Neuroimaging Modalities Using Deep Learning Techniques: Methods, Challenges, and Future Works. arXiv:210514278 (cs, eess] (Internet). (cited 2022 Feb 24); Available from: http://arxiv.org/ abs/2105.14278.
- Iasemidis, L.D., 2003. Epileptic seizure prediction and control. IEEE Transactions on Biomedical Engineering. 50(5), 549–558.
- Siddiqui, M.K., Morales-Menendez, R., Huang, X., et al., 2020. A review of epileptic seizure detection using machine learning classifiers. Brain Informatics. 7(1), 5.
- Hussein, A., Gomes, C., Habash, Q., et al., 2018. Focal and non-focal epilepsy localization: a review. IEEE Access. 6, 49306–49324.
- Nair, D.R., Laxer, K.D., Weber, P.B., et al., 2020. Nine-year prospective efficacy and safety of brain-responsive neurostimulation for focal epilepsy. Neurology. 95(9), e1244–e1256.
- Ngoh, A., Parker, A.P., 2017. New developments in epilepsy management. Paediatrics and Child Health. 27(6), 281–286.
- Rennie, J.M., 1997. Neonatal seizures. European Journal of Pediatrics. 156(2), 83–87.
- Moshé, S.L., 1993. Seizures in the developing brain. Neurology. 43(11 Suppl 5), S3-S7.
- Younkin, D.P., Delivoria-Papadopoulos, M., Maris, J., et al., 1986. Cerebral metabolic effects of neonatal seizures measured with in vivo 31P NMR spectroscopy. Annals of Neurology. 20(4), 513–519.
- Clancy, R.R., Legido, A., 1987. The exact ictal and interictal duration of electroencephalographic neonatal seizures. Epilepsia. 28(5), 537–541.
- Scher, M.S., Hamid, M.Y., Steppe, D.A., et al., 1993. Ictal and interictal electrographic seizure durations in preterm and term neonates. Epilepsia. 34(2), 284– 288.
- Tapani, K.T., Vanhatalo, S., Stevenson, N.J., 2019. Time-Varying EEG Correlations Improve Automated Neonatal Seizure Detection. International Journal of Neural Systems. 29(4), 1850030.
- Gilman, J.T., Gal, P., Duchowny, M.S., et al., 1989. Rapid sequential phenobarbital treatment of neonatal seizures. Pediatrics. 83(5), 674–678.
- Goldberg, H.J., 1983. Neonatal convulsions--a 10 year review. Archives of Disease in Childhood. 58(12), 976–978.
- Cheah, I.G.S., 2019. Economic assessment of neonatal intensive care. Translational Pediatrics. 8(3), 246–256.
- Nsugbe, E., 2021. A cybernetic framework for predicting preterm and enhancing care strategies: A review. Biomedical Engineering Advances. 2, 100024.
- Temko, A., Thomas, E., Marnane, W., et al., 2011. EEG-based neonatal seizure detection with Support Vector Machines. Clinical Neurophysiology. 122(3), 464–473.
- O’Shea, A., Lightbody, G., Boylan, G., et al., 2020. Neonatal seizure detection from raw multi-channel EEG using a fully convolutional architecture. Neural Networks. 123, 12–25.
- Stevenson, N.J., Tapani, K., Lauronen, L., et al., 2019. A dataset of neonatal EEG recordings with seizure annotations. Scientific Data. 6(1), 190039.
- Daly, A., O’Shea, A., Lightbody, G., et al., 2021. Towards Deeper Neural Networks for Neonatal Seizure Detection. Annual International Conference of the IEEE Engineering in Medicine and Biology Society. 920–923.
- Deburchgraeve, W., Cherian, P.J., De Vos, M., et al., 2008. Automated neonatal seizure detection mimicking a human observer reading EEG. Clinical Neurophysiology. 119(11), 2447–2454.
- Greene, B.R., Marnane, W.P., Lightbody, G., et al., 2008. Classifier models and architectures for EEGbased neonatal seizure detection. Physiological Measurement. 29(10), 1157–1178.
- Runarsson, T.P., Sigurdsson, S., 2005. On-line Detection of Patient Specific Neonatal Seizures using Support Vector Machines and Half-Wave Attribute Histograms. International Conference on Computational Intelligence for Modelling, Control and Automation and International Conference on Intelligent Agents, Web Technologies and Internet Commerce (CIMCA-IAWTIC’06). pp. 673–677.
- Aarabi, A., Grebe, R., Wallois, F., 2007. A multistage knowledge-based system for EEG seizure detection in newborn infants. Clinical Neurophysiology. 118(12), 2781–2797.
- Thomas, E.M., Temko, A., Marnane, W.P., et al., 2013. Discriminative and generative classification techniques applied to automated neonatal seizure detection. IEEE Journal of Biomedical and Health Informatics. 17(2), 297–304.
- Nsugbe, E., 2017. Particle size distribution estimation of a powder agglomeration process using acoustic emissions (Internet) (Thesis). (cited 2021 Dec 27). Available from: http://dspace.lib.cranfield.ac.uk/ handle/1826/14378.
- Nsugbe, E., Ruiz-Carcel, C., Starr, A., et al., 2018. Estimation of fine and oversize particle ratio in a heterogeneous compound with acoustic emissions. Sensors. 18(3), 851.
- Nsugbe, E., William Samuel, O., Asogbon, M.G., et al., 2021. Contrast of multi-resolution analysis approach to transhumeral phantom motion decoding. CAAI Transactions on Intelligence Technology. 6(3), 360–375.
- Nsugbe, E., Sanusi, I., 2021. Towards an affordable magnetomyography instrumentation and low model complexity approach for labour imminency prediction using a novel multiresolution analysis. Applied AI Letters (Internet). (cited 2021 Dec 27). 2(3). DOI: https://onlinelibrary.wiley.com/doi/10.1002/ail2.34
- Vanhatalo, S., Kaila, K., 2006. Development of neonatal EEG activity: from phenomenology to physiology. Seminars in Fetal and Neonatal Medicine. 11(6), 471–478.
- Friston, K.J., 1994. Functional and effective connectivity in neuroimaging: A synthesis. Human Brain Mapping. 2(1–2), 56–78.
- Omidvarnia, A., 2014. Newborn EEG connectivity analysis using time-frequency signal processing techniques (Internet) (PhD Thesis). The University of Queensland. (cited 2022 Feb 25). Available from: http://espace.library.uq.edu.au/view/UQ:341333.
- Neuroscience NewsNeuroscience News, 2017. Artificial Intelligence to Evaluate Brain Maturity in Preterm Infants (Internet). Neuroscience News. (cited 2022 Feb 25). Available from: https://neurosciencenews.com/ai-preterm-brain-7823/.
- Webb, L., Kauppila, M., Roberts, J.A., et al., 2021. Automated detection of artefacts in neonatal EEG with residual neural networks. Computer Methods and Programs in Biomedicine. 208, 106194.
- Nsugbe, E., Al-Timemy, A.H., Samuel, O.W. Intelligence combiner: a combination of deep learning and handcrafted features for an adolescent psychosis prediction using EEG signals. Unpublished results.
- Nsugbe, E., Samuel, O.W., Asogbon, M.G., et al., 2021. Phantom motion intent decoding for transhumeral prosthesis control with fused neuromuscular and brain wave signals. IET Cyber-Systems and Robotics. 3(1), 77–88.
- Nsugbe, E., Phillips, C., Fraser, M., et al., 2020. Gesture recognition for transhumeral prosthesis control using EMG and NIR. IET Cyber-Systems and Robotics. 2(3), 122–131.
- Nsugbe, E., Obajemu, O., Samuel, O.W., et al., 2021. Enhancing care strategies for preterm pregnancies by using a prediction machine to aid clinical care decisions. Machine Learning with Applications. 6, 100110.
- Molnar C. 5.2 Logistic Regression. Interpretable Machine Learning (Internet). (cited 2022 Feb 25). Available from: https://christophm.github.io/interpretable-ml-book/logistic.html.
- 1.10. Decision Trees (Internet). scikit-learn. (cited 2022 Feb 25). Available from: https://scikit-learn/stable/modules/tree.html.
- Noble, W.S., 2006. What is a support vector machine? Nature Biotechnology. 24(12), 1565–1567.
- Hopfield, J.J., 1988. Artificial neural networks. IEEE Circuits and Devices Magazine. 4(5), 3–10.
- Jinglin, Y., Li, H.X., Yong, H., 2011. A probabilistic SVM based decision system for pain diagnosis. Expert Systems with Applications. 38(8), 9346–9351.
- Böken, B., 2021. On the appropriateness of Platt scaling in classifier calibration. Information Systems. 95, 101641.
- Wang, J., Xu, M., Wang, H., et al., 2006. Classification of Imbalanced Data by Using the SMOTE Algorithm and Locally Linear Embedding. 2006 8th international Conference on Signal Processing.
- Birth Asphyxia - Seattle Children’s (Internet). Seattle Children’s Hospital. (cited 2022 Feb 25). Available from: https://www.seattlechildrens.org/conditions/birth-asphyxia/.
- Gillam-Krakauer, M., Gowen Jr, C.W., 2022. Birth Asphyxia. StatPearls (Internet). Treasure Island (FL): StatPearls Publishing (cited 2022 Feb 25). Available from:http://www.ncbi.nlm.nih.gov/books/NBK43078 2/.
- Malin, G.L., Morris, R.K., Khan, K.S., 2010. Strength of association between umbilical cord pH and perinatal and long term outcomes: systematic review and meta-analysis. British Medical Journal. 340, c1471.
- Urbanowicz, R.J., Meeker, M., La Cava, W., et al., 2018. Relief-based feature selection: Introduction and review. Journal of Biomedical Informatics. 85, 189–203.
- Spiewak, C., Islam, M.R., Assad-Uz-Zaman, M., et al., 2018. A Comprehensive Study on EMG Feature Extraction and Classifiers. Open Access Journal of Biomedical Engineering and its Applications.
- Chandrashekar, G., Sahin, F., 2014. A survey on feature selection methods. Computers & Electrical Engineering. 40(1), 16–28.