Article
On the Application of Metaheuristics and Deep Wavelet Scattering Decompositions for the Prediction of Adolescent Psychosis Using EEG Brain Wave Signals
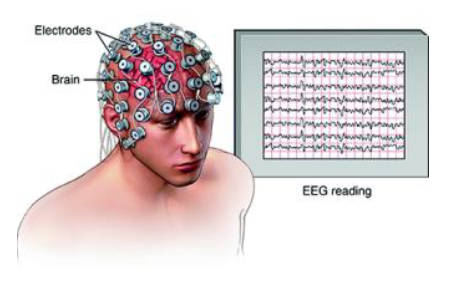
Downloads
Download

This work is licensed under a Creative Commons Attribution 4.0 International License.
Copyright
The authors shall retain the copyright of their work but allow the Publisher to publish, copy, distribute, and convey the work.
License
Digital Technologies Research and Applications (DTRA) publishes accepted manuscripts under Creative Commons Attribution 4.0 International (CC BY 4.0). Authors who submit their papers for publication by DTRA agree to have the CC BY 4.0 license applied to their work, and that anyone is allowed to reuse the article or part of it free of charge for any purpose, including commercial use. As long as the author and original source are properly cited, anyone may copy, redistribute, reuse, and transform the content.
Schizophrenia is a common psychotic disorder which affects a substantial amount of the population, where the paranoid variant is viewed as the most common form of the disorder. This form of psychosis has been seen to affect both adults and adolescents; where in the case of adolescents, it is increasingly challenging to diagnose with traditional means involving clinical interviews. The use of electroencephalography (EEG) signals has proven to be an effective means of non-invasively diagnosing brain disorders, alongside having the ability to mitigate any form of subjective bias from the diagnosis process. This paper explores the use of acquired EEG signals, metaheuristics and deep wavelet scattering decomposition, and a combination of supervised and unsupervised learning, for the automated prediction of adolescent schizophrenia. The results showed the best accuracy for the metaheuristic decomposition alongside the candidate learning methods, in the region of 95%+ across the various classification metrics, which showcases an enhanced means of prediction of adolescent schizophrenia. Further work would now explore the use of Long ShortTerm Memory and Convolution Neural Networks to investigate the classification performances.
Keywords:
Signal processing Evolutionary computing Pattern recognition Decision support EEG SchizophreniaReferences
- Arciniegas, D.B., 2015. Psychosis. Continuum (Minneap Minn). 21, 715-736. DOI: https://doi.org/10.1212/01.CON.0000466662.89908.e7
- Read, J., van Os, J., Morrison, A.P., et al., 2005. Childhood trauma, psychosis and schizophrenia: a literature review with theoretical and clinical implications. Acta Psychiatr Scand. 112, 330-350. DOI: https://doi.org/10.1111/j.1600-0447.2005.00634.x
- Prabhakar, S.K., Rajaguru, H., Kim, S.H., 2020. Schizophrenia EEG Signal Classification Based on Swarm Intelligence Computing. Computational Intelligence and Neuroscience. pp. e8853835. DOI: https://doi.org/10.1155/2020/8853835
- Gottesman, I.I., Shields, J., 1973. Genetic theorizing and schizophrenia. British Journal of Psychiatry. 122, 15-30. DOI: https://doi.org/10.1192/bjp.122.1.15
- World Health Organization (editor), 1992. The ICD10 classification of mental and behavioural disorders: clinical descriptions and diagnostic guidelines. Geneva: World Health Organization.
- Insel, T.R., 2010. Rethinking schizophrenia. Nature. 468, 187-193. DOI: https://doi.org/10.1038/nature09552
- Schmitt, A., Hasan, A., Gruber, O., et al., 2011. Schizophrenia as a disorder of disconnectivity. European Archives of Psychiatry and Clinical. 261, 150- 154. DOI: https://doi.org/10.1007/s00406-011-0242-2
- Devia, C., Mayol-Troncoso, R., Parrini, J., et al., 2019. EEG Classification During Scene Free-Viewing for Schizophrenia Detection. IEEE Transactions on Neural Systems & Rehabilitation. 27, 1193-1199. DOI: https://doi.org/10.1109/TNSRE.2019.2913799
- Hollis, C., 2000. Adolescent schizophrenia. Advances in Psychiatric Treatment. 6, 83-92. DOI: https://doi.org/10.1192/apt.6.2.83
- Gibbs, F.A., Gibbs, E.L., 1941. Atlas of electroencephalography. Oxford, England: F. A. Gibbs, Boston City Hospital.
- Darkhovsky, B., Piryatinska, A., Kaplan, A., 2016. Binary classification of multi-channel EEG records based on the ε-complexity of continuous vector functions. ArXiv:161001633 [Stat].
- Rickles, D., Hawe, P., Shiell, A., 2007. A simple guide to chaos and complexity. Journal of Epidemiology & Community Health. 61, 933-937. DOI: https://doi.org/10.1136/jech.2006.054254
- Arce, G.R., 2005. Nonlinear Signal Processing: A Statistical Approach. John Wiley & Sons.
- Nsugbe, E. Enhanced Recognition of Adolescent Schizophrenia and a Computational Contrast of their Neuroanatomy with Healthy Patients using Brain-wave Signals In peer review.
- Mallat, S.G., 1999. A wavelet tour of signal processing. 2nd ed. San Diego: Academic Press.
- Nsugbe, E., 2017. Particle size distribution estimation of a powder agglomeration process using acoustic emissions. PhD. Cranfield University.
- Addison, P.S., 2005. Wavelet transforms and the ECG: a review. Physiological Measurement. 26, R155-199. DOI: https://doi.org/10.1088/0967-3334/26/5/R01
- Sardy, S., Tseng, P., Bruce, A., 2001. Robust wavelet denoising. IEEE Transactions on Signal Processing. 49, 1146-1152. DOI: https://doi.org/10.1109/78.923297
- Adeli, H., Zhou, Z., Dadmehr, N., 2003. Analysis of EEG records in an epileptic patient using wavelet transform. Journal of Neuroscience Methods. 123, 69-87. DOI: https://doi.org/10.1016/S0165-0270(02)00340-0
- Bracewell, R.N., 2000. The Fourier transform and its applications. 3rd ed. Boston: McGraw Hill.
- Nsugbe, E., Starr, A., Foote, P., et al., 2016. Size differentiation of a continuous stream of particles using acoustic emissions. 20th Innovative Manufacturing Engineering and Energy Conference. 161, 012090. DOI: https://doi.org/10.1088/1757-899X/161/1/012090
- Nsugbe, E., Starr, A., Jennions, I., et al., 2017. Particle size distribution estimation of a mixture of regular and irregular sized particles using acoustic emissions. Procedia Manufacturing. 11, 2252-2259. DOI: https://doi.org/10.1016/j.promfg.2017.07.373
- Nsugbe, E., Ruiz-Carcel, C., Starr, A., et al., 2018. Estimation of fine and oversize particle ratio in a heterogeneous compound with acoustic emissions. Sensors. 18, 851.
- DOI: https://doi.org/10.3390/s18030851
- Nsugbe, E., Sanusi, I., 2021. Towards an affordable magnetomyography instrumentation and low model complexity approach for labour imminency prediction using a novel multiresolution analysis. Applied AI Letters. ail2, 34. DOI: https://doi.org/10.1002/ail2.34
- Nsugbe, E., Samuel, O.W., Asogbon, M.G., et al., 2021. Contrast of multi-resolution analysis approach to transhumeral phantom motion decoding. CAAI Transactions on Intelligence Technology. DOI: https://doi.org/10.1049/cit2.12039
- Albawi, S., Mohammed, T.A., Al-Zawi, S., 2017. Understanding of a convolutional neural network. 2017 International Conference on Engineering and Technology (ICET). pp. 1-6. DOI: https://doi.org/10.1109/ICEngTechnol.2017.8308186
- Andén, J., Mallat, S., 2014. Deep Scattering Spectrum. IEEE Transactions on Signal Processing. 62, 4114-4128. DOI: https://doi.org/10.1109/TSP.2014.2326991
- Khare, S., Bajaj, V., Siuly, S., et al., 2020. Classification of schizophrenia patients through empirical wavelet transformation using electroencephalogram signals. Modelling and Analysis of Active Biopotential Signals in Healthcare. IOP Science. 1, 1-26. DOI: https://doi.org/10.1088/978-0-7503-3279-8ch1
- Piryatinska, A., Darkhovsky, B., Kaplan, A., 2017. Binary classification of multichannel-EEG records based on the ϵ-complexity of continuous vector functions. Computer Methods and Programs in Biomedicine. 152, 131-139. DOI: https://doi.org/10.1016/j.cmpb.2017.09.001
- Prabhakar, S., Rajaguru, H., Kim, S.H., 2020. Schizophrenia EEG Signal Classification Based on Swarm Intelligence Computing. Computational Intelligence and Neuroscience. pp. 1-14. DOI: https://doi.org/10.1155/2020/8853835
- Sabeti, M., Boostani, R., Katebi, S.D., et al., 2007. Selection of relevant features for EEG signal classification of schizophrenic patients. Biomedical Signal Processing and Control. 2, 122-134. DOI: https://doi.org/10.1016/j.bspc.2007.03.003
- Prabhakar, S.K., Rajaguru, H., Lee, S.W., 2020. A Framework for Schizophrenia EEG Signal Classification With Nature Inspired Optimization Algorithms. IEEE Access. 8, 39875-39897. DOI: https://doi.org/10.1109/ACCESS.2020.2975848
- Kutepov, I.E., Dobriyan, V.V., Zhigalov, M.V., et al., 2020. EEG analysis in patients with schizophrenia based on Lyapunov exponents. Informatics in Medicine Unlocked. 18, 100289. DOI: https://doi.org/10.1016/j.imu.2020.100289
- Buettner, R., Beil, D., Scholtz, S., et al., 2020. Development of a Machine Learning Based Algorithm To Accurately Detect Schizophrenia based on One-minute EEG Recordings. DOI: https://doi.org/10.24251/HICSS.2020.393
- Parvinnia, E., Sabeti, M., Zolghadri Jahromi, M., et al., 2014. Classification of EEG Signals using adaptive weighted distance nearest neighbor algorithm. Journal of King Saud University - Computer and Information Sciences. 26, 1-6. DOI: https://doi.org/10.1016/j.jksuci.2013.01.001
- Sharma, M., Acharya, U.R., 2021. Automated detection of schizophrenia using optimal wavelet-based $$l_1$$ l 1 norm features extracted from single-channel EEG. Cognitive Neurodynamics. 15, 661-674. https://doi.org/10.1007/s11571-020-09655-w
- Darbas, M., Lohrengel, S., 2019. Review on Mathematical Modelling of Electroencephalography (EEG). Jahresbericht Der Deutschen Mathematiker. 121, 3-39. DOI: https://doi.org/10.1365/s13291-018-0183-z
- Siuly, S., Li, Y., Zhang, Y., 2016. Electroencephalogram (EEG) and Its Background. EEG Signal Analysis and Classification. pp. 3-21. DOI: https://doi.org/10.1007/978-3-319-47653-7_1
- EEG Database - Schizophrenia. MsuRu n.d. http:// brain.bio.msu.ru/eeg_schizophrenia.htm (Accessed October 10, 2021).
- Phinyomark, A., Hirunviriya, S., Limsakul, C., et al., 2010. Evaluation of EMG feature extraction for hand movement recognition based on Euclidean distance and standard deviation. ECTI-CON2010: The 2010 ECTI International Confernce on Electrical Engineering/Electronics, Computer, Telecommunications and Information Technology. pp. 856-860.
- Yu, X., Gen, M., 2010. Introduction to evolutionary algorithms. London ; New York: Springer.
- Bansal, S., Sharma, K., 2018. Nature-Inspired-Based Modified Multi-Objective BB-BC Algorithm to Find Near-OGRs for Optical WDM Systems and Its Performance Comparison. Handbook of Research on Biomimicry in Information Retrieval and Knowledge Management. pp. 1-25. DOI: https://doi.org/10.4018/978-1-5225-3004-6.ch001
- Bansal, S., Gupta, N., Singh, A.K., 2021. Application of Bat-Inspired Computing Algorithm and Its Variants in Search of Near-Optimal Golomb Rulers for WDM Systems: A Comparative Study. In: Dey N, Rajinikanth V, editors. Applications of Bat Algorithm and its Variants, Singapore: Springer. pp. 79-101. DOI: https://doi.org/10.1007/978-981-15-5097-3_5
- Wavelet Scattering. MathworksCom n.d. https://uk.mathworks.com/help/wavelet/ug/wavelet-scattering.html.
- Mallat, S., 2012. Group Invariant Scattering. Communications on Pure and Applied Mathematics. 65, 1331-1398. DOI: https://doi.org/10.1002/cpa.21413
- Bruna, J., Mallat, S., 2013. Invariant Scattering Convolution Networks. IEEE Transactions on Pattern Analysis and Machine Intelligence. 35, 1872-1886. DOI: https://doi.org/10.1109/TPAMI.2012.230
- Lostanlen, V., Scattering, M., 2021.
- Liu, Z., Yao, G., Zhang, Q., et al., 2020. Wavelet Scattering Transform for ECG Beat Classification. Computational and Mathematical Methods in Medicine. pp. 3215681.
- DOI: https://doi.org/10.1155/2020/3215681
- Andén, J., Mallat, S., 2011. Multiscale Scattering for Audio Classification. pp. 657-662.
- Nsugbe, E., Phillips, C., Fraser, M., et al., 2020. Gesture recognition for transhumeral prosthesis control using EMG and NIR. IET Cyber-Systems and Robotics. 2, 122-131.
- DOI: https://doi.org/10.1049/iet-csr.2020.0008
- Nsugbe, E., Samuel, O.W., Asogbon, M.G., et al., 2021. Phantom motion intent decoding for transhumeral prosthesis control with fused neuromuscular and brain wave signals. IET Cyber-Systems Robotics. 3, 77-88. DOI: https://doi.org/10.1049/csy2.12009
- Nsugbe, E., Obajemu, O., Samuel, O.W., et al., 2021. Application of noninvasive magnetomyography in labour imminency prediction for term and preterm pregnancies and ethnicity specific labour prediction. Machine Learning with Applications. 5, 100066.
- DOI: https://doi.org/10.1016/j.mlwa.2021.100066
- Nsugbe, E., Samuel, O.W., Asogbon, M.G., et al., 2020. A self-learning and adaptive control scheme for phantom prosthesis control using combined neuromuscular and brain-wave bio-signals. Engineering Proceedings. 2, 59. DOI: https://doi.org/10.3390/ecsa-7-08169
- Bochner, S., Chandrasekharan, K., 1950. Fourier Transforms. (AM-19). Princeton: Princeton University Press.
- Baradits, M., Kakuszi, B., Bálint, S., et al., 2019. Alterations in resting-state gamma activity in patients with schizophrenia: a high-density EEG study. European Archives of Psychiatry and Clinical Neuroscience. pp. 269. DOI: https://doi.org/10.1007/s00406-018-0889-z
- Janani, A.S., Grummett, T.S., Bakhshayesh, H., et al., 2018. How many channels are enough?: 26th European Signal Processing Conference, EUSIPCO 2018. 2018 26th European Signal Processing Conference, EUSIPCO 2018. pp. 101-105. DOI: https://doi.org/10.23919/EUSIPCO.2018.8553261