Communication
Fan-Beam Projection-Based Feature Extraction for Facial Expression Recognition
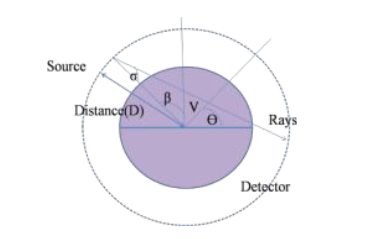
Downloads
Download


This work is licensed under a Creative Commons Attribution 4.0 International License.
Copyright
The authors shall retain the copyright of their work but allow the Publisher to publish, copy, distribute, and convey the work.
License
Digital Technologies Research and Applications (DTRA) publishes accepted manuscripts under Creative Commons Attribution 4.0 International (CC BY 4.0). Authors who submit their papers for publication by DTRA agree to have the CC BY 4.0 license applied to their work, and that anyone is allowed to reuse the article or part of it free of charge for any purpose, including commercial use. As long as the author and original source are properly cited, anyone may copy, redistribute, reuse, and transform the content.
This paper presents a novel method of feature extraction using Fan beam projection-based data. The Fanbeam projection covers the image completely and hence gathers all the important information. Even though the image quality is distorted, this type of feature extraction method helps to gather all the important information as there is a huge volume of projection data. Also, the use of multiple detectors speeds up the entire process. All the projections of the image together form a sinogram image which is unique for each facial expression image. Hence, the sinogram image is divided into grids and the histogram formation results in a feature vector for each image. The classification of these feature vectors using Radial Basis Function-based Extreme learning Machine (RBF-ELM) results in high classification accuracy for all the datasets.
Keywords:
Feature Expression Fan-beam Emotion Classification ImageReferences
- Kak, A.C., Slaney, M., 1988. Principles of Computerized Tomographic Imaging. IEEE Press, NY. pp. 92- 93.
- Lyons, M., Akamatsu, S., Kamachi, M., Gyoba, J., April 1998. Coding facial expressions with gabor wavelets. Third IEEE International Conference on Automatic Face and Gesture Recognition. Washington, DC, USA. pp. 200-205.
- Aifanti, N., Papachristou, C., Delopoulos, A., April 2010. The MUG facial expression database. 11th Int. Workshop on Image Analysis for Multimedia Interactive Services (WIAMIS), Italy. pp. 1-4.
- Pantic, M., Valstar, M., Rademaker, R., Maat, L., July 2005. Web-based database for facial expression analysis. In Multimedia and Expo, IEEE International Conference, Amsterdam. pp. 5.
- Valstar, M., Pantic, M., 2010. Induced disgust, happiness and surprise: an addition to the MMI facial expression database. In Proc. 3rd Intern. Workshop on EMOTION (satellite of LREC): Corpora for Research on Emotion and Affect. pp. 65.
- Dhall, A., Goecke, R., Lucey, S., Gedeon, T., 2012. Collecting large, richly annotated facial expression databases from movies. IEEE MultiMedia. 19, 34-41.
- Dhall, A., Goecke, R., Joshi, J., Sikka, K., Gedeon, T., 2014. Emotion recognition in the wild challenge 2014: Baseline, Data and Protocol. ACM ICMI.
- Zhao, G., Huang, X., Taini, M., Li, S.Z., Pietikäinen, M., 2011. Facial expression recognition from near-infrared videos. Image and Vision Computing. 29(9), 607-19.
- Ojala, T., Pietikainen, M., Maenpaa, T., 2002. Multiresolution gray-scale and rotation invariant texture classification with local binary patterns. IEEE Transactions on pattern analysis and machine intelligence. 24(7), 971-87.
- Jabid, T., Kabir, M.H., Chae, O., 2010. Robust facial expression recognition based on local directional pattern. ETRI journal. 32(5), 784-94.
- Tan, X., Triggs, B., 2010. Enhanced local texture feature sets for face recognition under difficult lighting conditions. IEEE transactions on image processing. 19(6), 1635-50.
- Alphonse, A.S., Dharma, D., 2018. Novel directional patterns and a Generalized Supervised Dimension Reduction System (GSDRS) for facial emotion recognition. Multimedia Tools and Applications. 77(8), 9455-88.
- Alphonse, A.S., Dharma, D., 2017. Enhanced Gabor (E-Gabor), Hypersphere-based normalization and Pearson General Kernel-based discriminant analysis for dimension reduction and classification of facial emotions. Expert Systems with Applications. 90, 127-45.
- Alphonse, A.S., Dharma, D., 2017. A novel Monogenic Directional Pattern (MDP) and pseudo-Voigt kernel for facilitating the identification of facial emotions. Journal of Visual Communication and Image Representation. 49, 459-70.
- Huang, G.B., Zhou, H., Ding, X., Zhang, R., 2012. Extreme learning machine for regression and multiclass classification. IEEE Transactions on Systems, Man, and Cybernetics. 42(2), 513-529.
- Chang, C.C., Lin, C.J., 2011. LIBSVM: a library for support vector machines. ACM Trans. Intell. Syst. Technology. 2(3), 27.
- Keyvanrad, M.A., Homayounpour, M., Aug. 2014. A brief survey on deep belief networks and introducing a new object oriented toolbox (DeeBNet). arXiv:1408.3264 [cs].