Article
Hybrid Operating Mode Management to Maximize the Service Life of Electrolyzers Running on Renewables
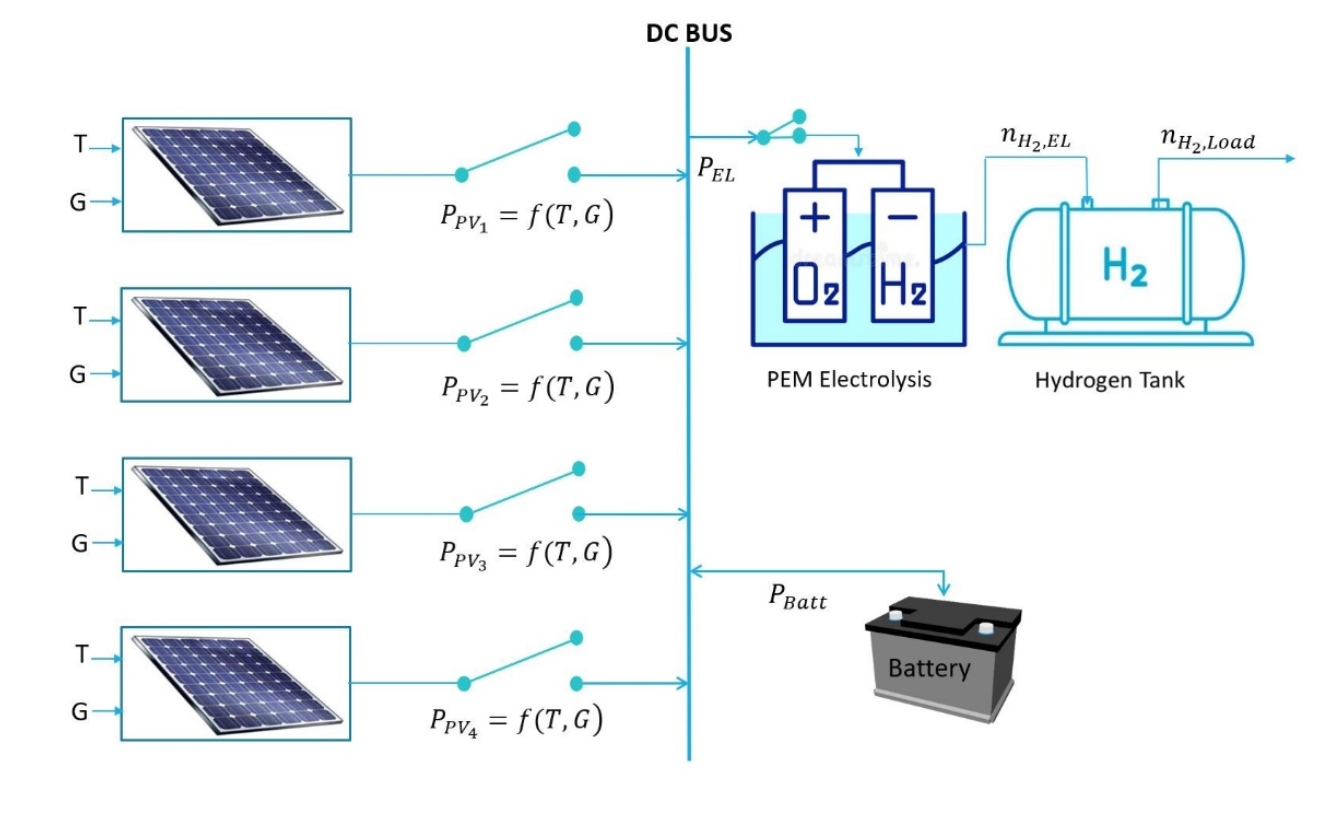
Downloads
Download

This work is licensed under a Creative Commons Attribution 4.0 International License.
Copyright
The authors shall retain the copyright of their work but allow the Publisher to publish, copy, distribute, and convey the work.
License
New Energy Exploitation and Application (NEEA) publishes accepted manuscripts under Creative Commons Attribution 4.0 International (CC BY 4.0). Authors who submit their papers for publication by New Energy Exploitation and Application (NEEA) agree to have the CC BY 4.0 license applied to their work, and that anyone is allowed to reuse the article or part of it free of charge for any purpose, including commercial use. As long as the author and original source is properly cited, anyone may copy, redistribute, reuse and transform the content.
The service life of equipment is generally linked to degradation factors depending on its operating conditions, including the rate of use and the frequency of the switching modes. The novel operating mode management proposed in this paper takes into account equipment lifetime in addition to all the previously mentioned requirements. This algorithm does not rely only on real-time data, as is traditionally presented in the literature, but also integrates predictive operating data. Therefore, it can be considered as a hybrid operating mode management as it embeds both predictive and event data, which yields improved results with respect to traditional event-driven management. This allows to optimize a criterion over a finite horizon, and, hence, an optimal sequence of the switching times of the different components of an energy system are generated. While the proposed approach is considered to be generic, it is illustrated by the production of green hydrogen from renewable sources. In order to ensure the operating safety and energy efficiency of the system, the objective is to maximize the life duration of the electrolyzer and the batteries by avoiding excessive stored quantities. Simulations using data obtained from a laboratory platform which replicates the process at a smaller scale highlight the effectiveness of the proposed approach.
Keywords:
Operating Modes Management; Hybrid-driven, Remaining Useful Lifetime; Optimization; Degradation Capacity; Green Hydrogen Production StationsReferences
- Chatti, N.; Gehin, A.-L.; Ould-Bouamama, B.; et al. Functional and behavior models for the supervision of an intelligent and autonomous system. IEEE Trans. Autom. Sci. Eng. 2013, 10, 431–445. DOI: https://doi.org/10.1109/TASE.2012.2211350
- Gehin, A.-L.; Staroswiecki, M. Reconfiguration analysis using generic component models. IEEE Trans. Syst. Man Cybern. A Syst. Hum. 2008, 38, 575–583.
- Watanabe, A.T.; Sebem, R.; Leal, A.B.; et al. Fault prognosis of discrete event systems: An overview. Annu. Rev. Control 2021, 51, 100–110.
- Arunthavanathan, R.; Khan, F.; Ahmed, S.; et al. An analysis of process fault diagnosis methods from safety perspectives. Comput. Chem. Eng. 2021, 145, 107197.
- Venkatasubramanian, V., Rengaswamy, R., Yin, K., et al. A review of process fault detection and diagnosis: Part i: Quantitative model-based methods. Comput. Chem. Eng. 2003, 27, 293–311. DOI: https://doi.org/10.1016/S0098-1354(02)00160-6
- Reiter, R. A theory of diagnosis from first principles. Artif. Intell. 1987, 32, 57–95. DOI: https://doi.org/10.1016/0004-3702(87)90062-2
- Pulido, B.; Gonzalez, C.A. Possible conflicts: a compilation technique for consistency-based diagnosis. IEEE Trans. Syst. Man Cybern. B Cybern. 2004, 34, 2192–2206.
- Meng, Q.; Yang, H.; Jiang, B.; et al. Accessibility, observability, and fault-tolerant control structure selection of network nonlinear systems. IEEE Trans. Control Netw. Syst. 2022, 9, 75–87.
- Trapiello, C.; Puig, V.; Cembrano, G. Reconfiguration of large-scale systems using back-up components. Comput. Chem. Eng. 2021, 149, 107288.
- Van der Schaft, A.J.; Schumacher, J.M. Modelling and analysis of hybrid dynamical systems. In Advances in the Control of Nonlinear Systems; Banos, A., Lamnabhi-Lagarrigue, F., Montoya, F.J., Eds.; Springer: London, UK, 2001; pp. 195–224. .
- Yu, M.; Xiao, C.; Zhang, B. Event-triggered discrete component prognosis of hybrid systems using degradation model selection. IEEE Trans. Ind. Electron. 2020, 68, 11470–11481.
- Connolly, C.; Taylor, K.; Bankhead, M.; et al. Techno-Economic Analysis of Heat-Assisted Hydrogen Production from Nuclear Power. New Energy Exploit. Appl. 2024, 3, 108–129. DOI: https://doi.org/10.54963/neea.v3i1.234
- Abdallah, I.; Gehin, A.-L.; Ould Bouamama, B. Event driven hybrid bond graph for hybrid renewable energy systems part i: Modelling and operating mode management. Int. J. Hydrogen Energy 2018, 43, 22088–22107. DOI: https://doi.org/10.1016/j.ijhydene.2017.10.144
- Krishnan, S., Koning, V., Theodorus de Groot, M.; et al. Present and future cost of alkaline and pem electrolyser stacks. Int. J. Hydrogen Energy 2023, 48, 32313–32330. DOI: https://doi.org/10.1016/j.ijhydene.2023.05.031
- Šimunović, J.; Radica, G.; Barbir, F. The effect of components capacity loss on the performance of a hybrid PV/wind/battery/hydrogen stand-alone energy system. Energy Convers. Manag. 2023, 291, 117314.
- Liberzon, D. Switching in Systems and Control; Birkhauser: Boston, MA, USA, 2012; pp. 1–12.
- Tuntas, R.; Hames, Y. Modeling of switching systems with artificial neural networks. J. Appl. Sci. 2005, 5, 1232–1236.
- Abdallah, I.; Gehin, A.-L.; Ould Bouamama, B. Event driven hybrid bond graph for diagnosis. In Proceedings of the 2016 European Control Conference, Aalborg, Denmark, 29 June–1 July 2016.
- Bouchebbat, R.; Gherbi, S. A novel optimal control and management strategy of stand-alone hybrid pv/wind/diesel power system. J. Control Autom. Electr. Syst. 2017, 28, 284–296.
- Sood, S.; Prakash, O.; Dieulot, J.Y.; et al. Robust diagnosis of PEM electrolysers using LFT bond graph. Int. J. Hydrogen Energy 2022, 47, 33938–33954.
- Sood, S.; Prakash, O.; Boukerdja, M.; et al. Generic dynamical model of PEM electrolyser under intermittent sources. Energies 2020, 13, 6556.
- Belkacem, L.; Simeu-Abazi, Z.; Dhouibi, H.; et al. Diagnostic and prognostic of hybrid dynamic systems: Modeling and RUL evaluation for two maintenance policies. Reliab. Eng. Syst. Saf. 2017, 164, 98–109. DOI: https://doi.org/10.1016/j.ress.2017.03.008
- El Kafazi, I.; Bannari, R.M. Multiobjective scheduling-based energy management system considering renewable energy and energy storage systems: a case study and experimental result. J. Control Autom. Electr. Syst. 2019, 30, 1030–1040. DOI: https://doi.org/10.1007/s40313-019-00524-4
- Ghane-Kanafi, A.; Khorram, E. A new scalarization method for finding the efficient frontier in non-convex multi-objective problems. Appl. Math. Modell. 2015, 39, 7483–7498. DOI: https://doi.org/10.1016/j.apm.2015.03.022
- Marler, R.T.; Arora, J.S. The weighted sum method for multi-objective optimization: new insights. Struct. Multidiscip. Optim. 2010, 41, 853–862. DOI: https://doi.org/10.1007/s00158-009-0460-7
- Dufo-López, R. Off-grid full renewable hybrid systems: Control strategies, optimization, and modeling. In Hybrid Technologies for Power Generation; Lo Faro, M., Barbera, O., Giacoppo, G., Eds.; Academic Press: Cambridge, MA, USA. pp. 69–100.
- Oliveira, D.Q.; Saavedra, O.R.; Santos-Pereira, K.; et al. A critical review of energy storage technologies for microgrids. Energy Syst. 2021, , 1–30.
- Nguyen, M.Y.; Nguyen, D.H.; Yoon, Y.T. A new battery energy storage charging/discharging scheme for wind power producers in real-time markets. Energies 2012, 5, 5439–5452. DOI: https://doi.org/10.3390/en5125439
- Santos-Pereira, K.; Pereira, J.D.; Veras, L.S.; et al. The requirements and constraints of storage technology in isolated microgrids: A comparative analysis of lithium-ion vs. lead-acid batteries. Energy Syst. 2021, 1–24. DOI: https://doi.org/10.1007/s12667-021-00439-7
- Schiffer, J.; Sauer, D.U.; Bindner, H.; et al. Model prediction for ranking lead-acid batteries according to expected lifetime in renewable energy systems and autonomous power-supply systems. J. Power Sources 2007, 168, 66–78. DOI: https://doi.org/10.1016/j.jpowsour.2006.11.092
- Bashir, N.; Sardar, H.S.; Nasir, M.; et al. Lifetime maximization of lead-acid batteries in small scale ups and distributed generation systems. In Proceedings of the 2017 IEEE Manchester PowerTech, Manchester, UK, 18–22 June 2017.
- Arsad, A.Z.; Hannan, M.A.; Al-Shetwi, A.Q.; et al. Hydrogen electrolyser technologies and their modelling for sustainable energy production: A comprehensive review and suggestions. Int. J. Hydrogen Energy 2023, 48, 27841–27871.
- Xu, B.; Ma, W.; Wu, W.; et al. Degradation Prediction of Pem Water Electrolyzer Under Constant and Start-Stop Loads Based on Cnn-Lstm. Energy AI 2024, 18, 100420.
- Chandesris, M.; Médeau, V.; Guillet, N.; et al. Membrane degradation in PEM water electrolyzer: Numerical modeling and experimental evidence of the influence of temperature and current density. Int. J. Hydrogen Energy 2015, 40, 1353–1366.
- Kuhnert, E.; Mayer, K.; Heidinger, M.; et al. Impact of intermittent operation on photovoltaic-PEM electrolyzer systems: A degradation study based on accelerated stress testing. Int. J. Hydrogen Energy 2024, 55, 683–695.
- Abdallah, R.; Gehin, A.-L.; Dieulot, J.-Y. Battery lifetime optimization in a solar microgrid. In Proceedings of the 2022 IEEE 17th international conference on control and automation, Naples, Italy, 27–30 June 2022.
- Jangdoost, A.; Keypour, R.; Golmohamadi, H. Optimization of distribution network reconfiguration by a novel RCA integrated with genetic algorithm. Energy Syst. 2021, 12, 801–833. DOI: https://doi.org/10.1007/s12667-020-00398-5