Article
Developing Artificial Neural Networks and Highway Safety Manual Models for Predicting Accidents at Intersections in Bahrain
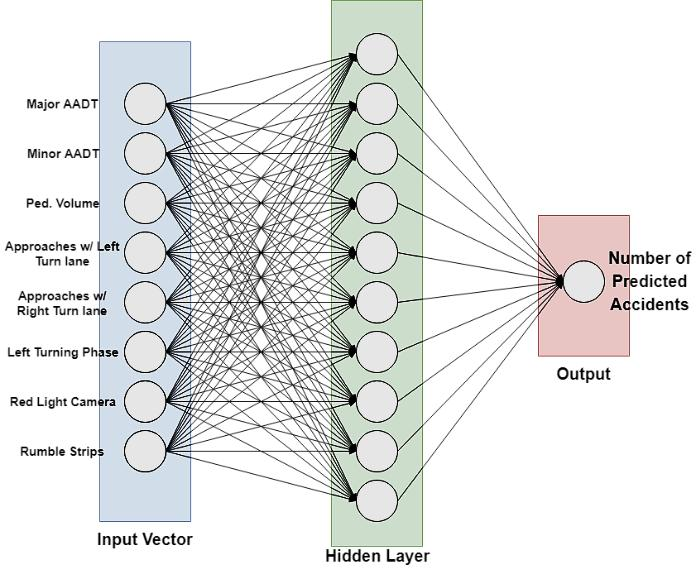
Downloads
Download

This work is licensed under a Creative Commons Attribution 4.0 International License.
Copyright
The authors shall retain the copyright of their work but allow the Publisher to publish, copy, distribute, and convey the work.
License
Digital Technologies Research and Applications (DTRA) publishes accepted manuscripts under Creative Commons Attribution 4.0 International (CC BY 4.0). Authors who submit their papers for publication by DTRA agree to have the CC BY 4.0 license applied to their work, and that anyone is allowed to reuse the article or part of it free of charge for any purpose, including commercial use. As long as the author and original source are properly cited, anyone may copy, redistribute, reuse, and transform the content.
Intersections are among the places where the highest number of accidents occur, thus, studying their safety and considering countermeasures to increase their safety should improve the overall safety of a traffic system. Prediction models, such as Artificial Neural Networks, have not been used for planning purposes in terms of providing countermeasures for accidents. This shortcoming forces the practitioners to employ traditional statistical methods which may be less accurate and have restricted applications. Hence, the Artificial Neural Networks models of this study were developed with the application of suggested countermeasures. Their performance was also compared with the traditional method given in the Highway Safety Manual after calibrating the procedure for local conditions. In this study, the intersections with the highest reported accidents isn the Kingdom of Bahrain were analyzed. The data was taken for the years 2013–2016, courtesy of the data provided by the Bahrain General Directorate of Traffic. Using this data, two predictive Artificial Neural Networks models were developed and used to forecast the accident number and severity in these selected intersections. Four intersections were selected to showcase the findings and to study the potential countermeasures that can be applied to reduce the occurrence of accidents. The comparison between Artificial Neural Networks and Highway Safety Manual procedures showed that Artificial Neural Networks models were more convenient to use with generic applications to different types of intersections. Moreover, they also provided higher accuracy while the Highway Safety Manual model was found to be heavily dependent upon traffic demand, which greatly affected its accuracy. The countermeasures suggested in this study were shown to reduce the accidents at the selected locations.
Keywords:
traffic; car accidents; artificial neural network; accident predictionReferences
- Witten, I.H.; Frank, E.; Hall, M.A.; Pal, C.J. Data Mining: Practical Machine Learning Tools and Techniques, 3rd ed. Morgan Kaufmann: Burlington, Massachusetts, USA, 2011, 76–77.
- Bishop, C.M. Pattern Recognition and Machine Learning. Springer: New York, NY, USA, 17 August 2006, 738–739.
- Shopper analytics: A Customer Activity Recognition System Using a Distributed RGB-d Camera Network. International Workshop on Video Analytics for Audience Measurement in Retail and Digital Signage. Available online: https://arxiv.org/abs/1508.06853 (accessed on 23 May 2024).
- Aydrus, I.A.; Ryazantsev, S.V.; Bogdanov, I.Y.; Asmyatullin, R.R.; Homsi, M. The Role of Labor Resources in the Development of the Bahrain Economy. Amazonia Investiga 2019, 8, 95–106.
- Alaali, F.; Naser, H. Economic Development and Environmental Sustainability: Evidence from Bahrain. Energy Ecol. Environ. 2020, 5, 211–219.
- Zhang, G.; Yau, K.K.; Zhang, X.; Li, Y. Traffic Accidents Involving Fatigue Driving and Their Extent of Casualties. Accid. Anal. Prev. 2016, 87, 34–42.
- Gazder, U.; Ahmed, A.; Abdulhusain, B.H.; Mohamed, A.H.; Ratrout, N. Estimating the Severity Levels of Road Traffic Crashes in Bahrain with Crash Costs Estimated with Different Approaches. Digital Transp. Saf. 2023, 2, 278–283.
- Motamedi, M.H.K.; Dadgar, E.; Ebrahimi, A. Curbing Road Traffic Accidents–the Major Cause of Facial Fractures. Int. J. Emergency Mental Health 2014, 16, 326–327.
- Yasin Çodur, M.; Tortum, A. An Artificial Neural Network Model for Highway Accident Prediction: a Case Study of Erzurum, Turkey. PROMET-Traffic Transp. 2015, 27, 217–225.
- Alkheder, S.; Taamneh, M.; Taamneh, S. Severity Prediction of Traffic Accident Using an Artificial Neural Network. J. Forecasting 2017, 36, 100–108.
- Haghighat, A.K.; Ravichandra-Mouli, V.; Chakraborty, P.; Esfandiari, Y.; Arabi, S.; Sharma, A. Applications of Deep Learning in Intelligent Transportation Systems. J.Big Data Analytics Transp. 2020, 2, 115–145.
- Dong, C.; Shao, C.; Li, J.; Xiong, Z. An Improved Deep Learning Model for Traffic Crash Prediction. J. Adv. Transp. 2018, 1–13.
- Ali, Y.; Hussain, F.; Haque, M.M. Advances, Challenges, and Future Research Needs in Machine Learning-Based Crash Prediction Models: a Systematic Review. Accid. Anal. Prev. 2024, 194, 107378.
- Shinar, D.; Hauer, E. Crash Causation, Countermeasures, and Policy–Editorial. Accid. Anal. Prev. 2024, 201, 107543.
- AASHTO. Highway Safety Manual. Washington, DC 20001: American Association of State Highway and Transportation Official, 2010. Available online: https://www.scribd.com/document/678082230/AASHTO-Highway-Safety-Manual-1E-2010 (accessed on 23 May 2024)
- Sun, S.; Brown, H.; Edara, P.; Claros, B.; Nam, K. Calibration of the Highway Safety Manual for Missouri (No. MATC-MU: 177). Mid-America Transportation Center, 2013.Available online: https://digitalcommons.unl.edu/matcreports/94/ (accessed on 23 May 2024)
- La Torre, F.; Domenichini, L.; Corsi, F.; Fanfani, F. Transferability of the Highway Safety Manual Freeway Model to the Italian Motorway Network. Transp. Res. Rec. 2014, 2435, 61–71.
- Gupta, P.K. GWAS for Genetics of Complex Quantitative Traits: Genome to Pangenome and SNPs to SVs and k‐mers. BioEssays 2021, 43, 2100109.
- Pusuluri, V.L.; Dangeti, M.R.; Kotamrazu, M. Road Crash Zone Identification and Remedial Measures Using GIS. Innovative Infrastruct. Solutions 2023, 8, 146.
- Zhang, M.; Han, Y.; Zalhaf, A.S.; Wang, C.; Yang, P.; Wang, C.; Zhou, S.; Xiong, T. Accurate Ultra-short-term Load Forecasting Based on Load Characteristic Decomposition and Convolutional Neural Network with Bidirectional Long Short-Term Memory Model. Sustain Energy Grids 2023, 35, 101129.
- Ahmed, M.; Chalise, R. Calibration of the Highway Safety Manual’s Safety Performance Functions for Rural Two-Lane Highways with Regional Considerations for the Rocky Mountains and Plain Regions (No. MPC 18-344), 2018. Available online: https://www.ugpti.org/resources/reports/downloads/mpc18-344.pdf (accessed on 23 May 2024)
- Moraldi, F.; La Torre, F.; Ruhl, S. Transfer of the Highway Safety Manual Predictive Method to German Rural Two-lane, Two-way roads. J. Transp. Saf. Secur. 2019, 12, 977–996.
- GDT. Traffic Accident Facts. General Directorate of Traffic Services, 2013. Available online: https://crashstats.nhtsa.dot.gov/Api/Public/ViewPublication/812169 (accessed on 23 May 2024)
- GDT. Traffic Accident Facts. General Directorate of Traffic Services, 2014. Available online: https://crashstats.nhtsa.dot.gov/Api/Public/ViewPublication/812263 (accessed on 23 May 2024)
- GDT. Traffic Accident Facts. General Directorate of Traffic Services, 2015. Available online: https://crashstats.nhtsa.dot.gov/Api/Public/Publication/812412 (accessed on 23 May 2024)
- GDT. Traffic Accident Facts. General Directorate of Traffic Services, 2016. Available online: https://crashstats.nhtsa.dot.gov/Api/Public/Publication/812480 (accessed on 23 May 2024)
- Tahir, F.; Arshad, M.Y.; Saeed, M.A.; Ali, U. Integrated Process for Simulation of Gasification and Chemical Looping Hydrogen Production Using Artificial Neural Network and Machine Learning Validation. Energy Convers. Manage. 2023, 296, 117702.
- Adebiyi, A.A.; Adewumi, A.O.; Ayo, C.K. Comparison of ARIMA and Artificial Neural Networks Models for Stock Price Prediction. Eur. J. Appl. Math. 2014, 614342.
- Abdel-Hamid, O.; Mohamed, A.R.; Jiang, H.; Deng, L.; Penn, G.; Yu, D. Convolutional Neural Networks for Speech Recognition. IEEE/ACM Trans. Audio Speech Lang. Process. 2014, 22, 1533–1545.
- Seo, S.; Jo, S.H.; Kim, S.; Shim, J.; Oh, S.; Kim, J.H.;Heo, K.; Choi, J.-w.; Choi, C.; Oh, S., et al. Artificial Optic-neural Synapse for Colored and Color-mixed Pattern Recognition. Nat. Commun. 2018, 9, 5106.
- Zissis, D.; Xidias, E.K.; Lekkas, D. Real-time Vessel Behavior Prediction. Evolving Syst. 2016, 7, 29–40.
- Mirikitani, D.T.; Nikolaev, N. Recursive Bayesian Recurrent Neural Networks for Time-series Modeling. IEEE Trans. Neural Networks 2009, 21, 262–274.
- Retting, R.A.; Ferguson, S.A.; Hakkert, A.S. Effects of Red Light Cameras on Violations and Crashes: a Review of the International Literature. Traffic Inj. Prev. 2003, 4, 17–23.
- Thomas, A.; Hess, S. Red‐light Cameras for the Prevention of Road Traffic Crashes. Cochrane Database Syst. Rev. 2005, 2, CD003862.
- Stopping Behavior at Real-World Stop-Controlled Intersections with and without In-Lane Rumble Strips. Available online: https://conservancy.umn.edu/items/d054af6e-f93b-4397-b611-da95eb345357 (accessed on 15 July 2019).
- Long, K.; Liu, Y.; Han, L.D. Impact of Countdown Timer on Driving Maneuvers after the Yellow Onset at Signalized Intersections: An Empirical Study in Changsha, China. Saf. Sci. 2013, 54, 8–16.
- Monteiro, N.M.; Balogun, S.K.; Kote, M,; Tlhabano, K. Stationary Tailgating in Gaborone, Botswana: the Influence of Gender, Time of Day, Type of Vehicle and Presence of Traffic Officer. IATSS Res. 2015, 38, 157–163.
- Traffic engineering 4th Edition. Available online: https://www.academia.edu/94819708/Traffic_engineering_4th_Edition (accessed on 23 May 2024)
- Gazder, U.; Hasan, A.; Yousif, Y. Safety Evaluation of Intersections in Bahrain. In 2019 8th International Conference on Modeling Simulation and Applied Optimization (ICMSAO), Manama, Bahrain, 15–17 April 2019.